由于文章较长,所以我还是先把目录提前。
一、认识管道流
1.1 数据导入
1.2 使用管道创建工作流
二、K折交叉验证
2.1 K折交叉验证原理
2.2 K折交叉验证实现
三、曲线调参
3.1 模型准确度
3.2绘制学习曲线得到样本数与准确率的关系
3.3绘制验证曲线得到超参和准确率关系
四、网格搜索
4.1两层for循环暴力检索
4.2构建字典暴力检索
五、嵌套交叉验证
六、相关评价指标
6.1 混淆矩阵及其实现
6.2 相关评价指标实现
6.3 ROC曲线及其实现
一、认识管道流
今天先介绍一下管道工作流的操作。
“管道工作流”这个概念可能有点陌生,其实可以理解为一个容器,然后把我们需要进行的操作都封装在这个管道里面进行操作,比如数据标准化、特征降维、主成分分析、模型预测等等,下面还是以一个实例来讲解。
1.1 数据导入与预处理
本次我们导入一个二分类数据集 Breast Cancer Wisconsin,它包含569个样本。首列为主键ID,第2列为类别值(M=恶性肿瘤,B=良性肿瘤),第3-32列是实数值的特征。
先导入数据集:
1#导入相关数据集 2importpandasaspd 3importurllib 4try: 5df=pd.read_csv('https://archive.ics.uci.edu/ml/machine-learning-databases' 6'/breast-cancer-wisconsin/wdbc.data',header=None) 7excepturllib.error.URLError: 8df=pd.read_csv('https://raw.githubusercontent.com/rasbt/' 9'python-machine-learning-book/master/code/' 10'datasets/wdbc/wdbc.data',header=None) 11print('rows,columns:',df.shape) 12df.head()
使用我们学习过的LabelEncoder来转化类别特征:
1fromsklearn.preprocessingimportLabelEncoder 2X=df.loc[:,2:].values 3y=df.loc[:,1].values 4le=LabelEncoder() 5#将目标转为0-1变量 6y=le.fit_transform(y) 7le.transform(['M','B'])
划分训练验证集:
1##创建训练集和测试集 2fromsklearn.model_selectionimporttrain_test_split 3X_train,X_test,y_train,y_test= 4train_test_split(X,y,test_size=0.20,random_state=1)
1.2 使用管道创建工作流
很多机器学习算法要求特征取值范围要相同,因此需要对特征做标准化处理。此外,我们还想将原始的30维度特征压缩至更少维度,这就需要用到主成分分析,要用PCA来完成,再接着就可以进行logistic回归预测了。
Pipeline对象接收元组构成的列表作为输入,每个元组第一个值作为变量名,元组第二个元素是sklearn中的transformer或Estimator。管道中间每一步由sklearn中的transformer构成,最后一步是一个Estimator。
本次数据集中,管道包含两个中间步骤:StandardScaler和PCA,其都属于transformer,而逻辑斯蒂回归分类器属于Estimator。
本次实例,当管道pipe_lr执行fit方法时:
1)StandardScaler执行fit和transform方法;
2)将转换后的数据输入给PCA;
3)PCA同样执行fit和transform方法;
4)最后数据输入给LogisticRegression,训练一个LR模型。
对于管道来说,中间有多少个transformer都可以。管道的工作方式可以用下图来展示(一定要注意管道执行fit方法,而transformer要执行fit_transform):
上面的代码实现如下:
1fromsklearn.preprocessingimportStandardScaler#用于进行数据标准化 2fromsklearn.decompositionimportPCA#用于进行特征降维 3fromsklearn.linear_modelimportLogisticRegression#用于模型预测 4fromsklearn.pipelineimportPipeline 5pipe_lr=Pipeline([('scl',StandardScaler()), 6('pca',PCA(n_components=2)), 7('clf',LogisticRegression(random_state=1))]) 8pipe_lr.fit(X_train,y_train) 9print('TestAccuracy:%.3f'%pipe_lr.score(X_test,y_test)) 10y_pred=pipe_lr.predict(X_test)
Test Accuracy: 0.947
二、K折交叉验证
为什么要评估模型的泛化能力,相信这个大家应该没有疑惑,一个模型如果性能不好,要么是因为模型过于复杂导致过拟合(高方差),要么是模型过于简单导致导致欠拟合(高偏差)。如何评估它,用什么数据来评估它,成为了模型评估需要重点考虑的问题。
我们常规做法,就是将数据集划分为3部分,分别是训练、测试和验证,彼此之间的数据不重叠。但,如果我们遇见了数据量不多的时候,这种操作就显得不太现实,这个时候k折交叉验证就发挥优势了。
2.1 K折交叉验证原理
先不多说,先贴一张原理图(以10折交叉验证为例)。
k折交叉验证步骤:
Step 1:使用不重复抽样将原始数据随机分为k份;
Step2:其中k-1份数据用于模型训练,剩下的那1份数据用于测试模型;
Step 3:重复Step 2k次,得到k个模型和他的评估结果。
Step 4:计算k折交叉验证结果的平均值作为参数/模型的性能评估。
2.1 K折交叉验证实现
K折交叉验证,那么K的取值该如何确认呢?一般我们默认10折,但根据实际情况有所调整。我们要知道,当K很大的时候,你需要训练的模型就会很多,这样子对效率影响较大,而且每个模型的训练集都差不多,效果也差不多。我们常用的K值在5~12。
我们根据k折交叉验证的原理步骤,在sklearn中进行10折交叉验证的代码实现:
1importnumpyasnp 2fromsklearn.model_selectionimportStratifiedKFold 3kfold=StratifiedKFold(n_splits=10, 4random_state=1).split(X_train,y_train) 5scores=[] 6fork,(train,test)inenumerate(kfold): 7pipe_lr.fit(X_train[train],y_train[train]) 8score=pipe_lr.score(X_train[test],y_train[test]) 9scores.append(score) 10print('Fold:%s,Classdist.:%s,Acc:%.3f'%(k+1, 11np.bincount(y_train[train]),score)) 12print(' CVaccuracy:%.3f+/-%.3f'%(np.mean(scores),np.std(scores)))
output:
当然,实际使用的时候没必要这样子写,sklearn已经有现成封装好的方法,直接调用即可。
1fromsklearn.model_selectionimportcross_val_score 2scores=cross_val_score(estimator=pipe_lr, 3X=X_train, 4y=y_train, 5cv=10, 6n_jobs=1) 7print('CVaccuracyscores:%s'%scores) 8print('CVaccuracy:%.3f+/-%.3f'%(np.mean(scores),np.std(scores)))
三、曲线调参
我们讲到的曲线,具体指的是学习曲线(learning curve)和验证曲线(validation curve)。
3.1 模型准确率(Accuracy)
模型准确率反馈了模型的效果,大家看下图:
1)左上角子的模型偏差很高。它的训练集和验证集准确率都很低,很可能是欠拟合。解决欠拟合的方法就是增加模型参数,比如,构建更多的特征,减小正则项。
2)右上角子的模型方差很高,表现就是训练集和验证集准确率相差太多。解决过拟合的方法有增大训练集或者降低模型复杂度,比如增大正则项,或者通过特征选择减少特征数。
3)右下角的模型就很好。
3.2 绘制学习曲线得到样本数与准确率的关系
直接上代码:
1importmatplotlib.pyplotasplt 2fromsklearn.model_selectionimportlearning_curve 3pipe_lr=Pipeline([('scl',StandardScaler()), 4('clf',LogisticRegression(penalty='l2',random_state=0))]) 5train_sizes,train_scores,test_scores= 6learning_curve(estimator=pipe_lr, 7X=X_train, 8y=y_train, 9train_sizes=np.linspace(0.1,1.0,10),#在0.1和1间线性的取10个值 10cv=10, 11n_jobs=1) 12train_mean=np.mean(train_scores,axis=1) 13train_std=np.std(train_scores,axis=1) 14test_mean=np.mean(test_scores,axis=1) 15test_std=np.std(test_scores,axis=1) 16plt.plot(train_sizes,train_mean, 17color='blue',marker='o', 18markersize=5,label='trainingaccuracy') 19plt.fill_between(train_sizes, 20train_mean+train_std, 21train_mean-train_std, 22alpha=0.15,color='blue') 23plt.plot(train_sizes,test_mean, 24color='green',linestyle='--', 25marker='s',markersize=5, 26label='validationaccuracy') 27plt.fill_between(train_sizes, 28test_mean+test_std, 29test_mean-test_std, 30alpha=0.15,color='green') 31plt.grid() 32plt.xlabel('Numberoftrainingsamples') 33plt.ylabel('Accuracy') 34plt.legend(loc='lowerright') 35plt.ylim([0.8,1.0]) 36plt.tight_layout() 37plt.show()
Learning_curve中的train_sizes参数控制产生学习曲线的训练样本的绝对/相对数量,此处,我们设置的train_sizes=np.linspace(0.1, 1.0, 10),将训练集大小划分为10个相等的区间,在0.1和1之间线性的取10个值。learning_curve默认使用分层k折交叉验证计算交叉验证的准确率,我们通过cv设置k。
下图可以看到,模型在测试集表现很好,不过训练集和测试集的准确率还是有一段小间隔,可能是模型有点过拟合。
3.3 绘制验证曲线得到超参和准确率关系
验证曲线是用来提高模型的性能,验证曲线和学习曲线很相近,不同的是这里画出的是不同参数下模型的准确率而不是不同训练集大小下的准确率:
1fromsklearn.model_selectionimportvalidation_curve 2param_range=[0.001,0.01,0.1,1.0,10.0,100.0] 3train_scores,test_scores=validation_curve( 4estimator=pipe_lr, 5X=X_train, 6y=y_train, 7param_name='clf__C', 8param_range=param_range, 9cv=10) 10train_mean=np.mean(train_scores,axis=1) 11train_std=np.std(train_scores,axis=1) 12test_mean=np.mean(test_scores,axis=1) 13test_std=np.std(test_scores,axis=1) 14plt.plot(param_range,train_mean, 15color='blue',marker='o', 16markersize=5,label='trainingaccuracy') 17plt.fill_between(param_range,train_mean+train_std, 18train_mean-train_std,alpha=0.15, 19color='blue') 20plt.plot(param_range,test_mean, 21color='green',linestyle='--', 22marker='s',markersize=5, 23label='validationaccuracy') 24plt.fill_between(param_range, 25test_mean+test_std, 26test_mean-test_std, 27alpha=0.15,color='green') 28plt.grid() 29plt.xscale('log') 30plt.legend(loc='lowerright') 31plt.xlabel('ParameterC') 32plt.ylabel('Accuracy') 33plt.ylim([0.8,1.0]) 34plt.tight_layout() 35plt.show()
我们得到了参数C的验证曲线。和learning_curve方法很像,validation_curve方法使用采样k折交叉验证来评估模型的性能。在validation_curve内部,我们设定了用来评估的参数(这里我们设置C作为观测)。
从下图可以看出,最好的C值是0.1。
四、网格搜索
网格搜索(grid search),作为调参很常用的方法,这边还是要简单介绍一下。
在我们的机器学习算法中,有一类参数,需要人工进行设定,我们称之为“超参”,也就是算法中的参数,比如学习率、正则项系数或者决策树的深度等。
网格搜索就是要找到一个最优的参数,从而使得模型的效果最佳,而它实现的原理其实就是暴力搜索;即我们事先为每个参数设定一组值,然后穷举各种参数组合,找到最好的那一组。
4.1. 两层for循环暴力检索
网格搜索的结果获得了指定的最优参数值,c为100,gamma为0.001
1#naivegridsearchimplementation 2fromsklearn.datasetsimportload_iris 3fromsklearn.svmimportSVC 4fromsklearn.model_selectionimporttrain_test_split 5iris=load_iris() 6X_train,X_test,y_train,y_test=train_test_split(iris.data,iris.target,random_state=0) 7print("Sizeoftrainingset:%dsizeoftestset:%d"%(X_train.shape[0],X_test.shape[0])) 8best_score=0 9forgammain[0.001,0.01,0.1,1,10,100]: 10forCin[0.001,0.01,0.1,1,10,100]: 11#foreachcombinationofparameters 12#trainanSVC 13svm=SVC(gamma=gamma,C=C) 14svm.fit(X_train,y_train) 15#evaluatetheSVConthetestset 16score=svm.score(X_test,y_test) 17#ifwegotabetterscore,storethescoreandparameters 18ifscore>best_score: 19best_score=score 20best_parameters={'C':C,'gamma':gamma} 21print("bestscore:",best_score) 22print("bestparameters:",best_parameters)
output: Size of training set: 112 size of test set: 38 best score: 0.973684210526 best parameters: {'C': 100, 'gamma': 0.001}
4.2. 构建字典暴力检索
网格搜索的结果获得了指定的最优参数值,c为1
1fromsklearn.svmimportSVC 2fromsklearn.model_selectionimportGridSearchCV 3pipe_svc=Pipeline([('scl',StandardScaler()), 4('clf',SVC(random_state=1))]) 5param_range=[0.0001,0.001,0.01,0.1,1.0,10.0,100.0,1000.0] 6param_grid=[{'clf__C':param_range, 7'clf__kernel':['linear']}, 8{'clf__C':param_range, 9'clf__gamma':param_range, 10'clf__kernel':['rbf']}] 11gs=GridSearchCV(estimator=pipe_svc, 12param_grid=param_grid, 13scoring='accuracy', 14cv=10, 15n_jobs=-1) 16gs=gs.fit(X_train,y_train) 17print(gs.best_score_) 18print(gs.best_params_)
output: 0.978021978022 {'clf__C': 0.1, 'clf__kernel': 'linear'}
GridSearchCV中param_grid参数是字典构成的列表。对于线性SVM,我们只评估参数C;对于RBF核SVM,我们评估C和gamma。最后, 我们通过best_parmas_得到最优参数组合。
接着,我们直接利用最优参数建模(best_estimator_):
1clf=gs.best_estimator_ 2clf.fit(X_train,y_train) 3print('Testaccuracy:%.3f'%clf.score(X_test,y_test))
网格搜索虽然不错,但是穷举过于耗时,sklearn中还实现了随机搜索,使用 RandomizedSearchCV类,随机采样出不同的参数组合。
五、嵌套交叉验证
嵌套交叉验证(nested cross validation)选择算法(外循环通过k折等进行参数优化,内循环使用交叉验证),对特定数据集进行模型选择。Varma和Simon在论文Bias in Error Estimation When Using Cross-validation for Model Selection中指出使用嵌套交叉验证得到的测试集误差几乎就是真实误差。
嵌套交叉验证外部有一个k折交叉验证将数据分为训练集和测试集,内部交叉验证用于选择模型算法。
下图演示了一个5折外层交叉沿则和2折内部交叉验证组成的嵌套交叉验证,也被称为5*2交叉验证:
我们还是用到之前的数据集,相关包的导入操作这里就省略了。
SVM分类器的预测准确率代码实现:
1gs=GridSearchCV(estimator=pipe_svc, 2param_grid=param_grid, 3scoring='accuracy', 4cv=2) 5 6#Note:Optionally,youcouldusecv=2 7#intheGridSearchCVabovetoproduce 8#the5x2nestedCVthatisshowninthefigure. 9 10scores=cross_val_score(gs,X_train,y_train,scoring='accuracy',cv=5) 11print('CVaccuracy:%.3f+/-%.3f'%(np.mean(scores),np.std(scores)))
CV accuracy: 0.965 +/- 0.025
决策树分类器的预测准确率代码实现:
1fromsklearn.treeimportDecisionTreeClassifier 2 3gs=GridSearchCV(estimator=DecisionTreeClassifier(random_state=0), 4param_grid=[{'max_depth':[1,2,3,4,5,6,7,None]}], 5scoring='accuracy', 6cv=2) 7scores=cross_val_score(gs,X_train,y_train,scoring='accuracy',cv=5) 8print('CVaccuracy:%.3f+/-%.3f'%(np.mean(scores),np.std(scores)))
CV accuracy: 0.921 +/- 0.029
六、相关评价指标
6.1 混淆矩阵及其实现
混淆矩阵,大家应该都有听说过,大致就是长下面这样子的:
所以,有几个概念需要先说明:
TP(True Positive): 真实为0,预测也为0
FN(False Negative): 真实为0,预测为1
FP(False Positive): 真实为1,预测为0
TN(True Negative): 真实为1,预测也为1
所以,衍生了几个常用的指标:
: 分类模型总体判断的准确率(包括了所有class的总体准确率)
: 预测为0的准确率
: 真实为0的准确率
: 真实为1的准确率
: 预测为1的准确率
:对于某个分类,综合了Precision和Recall的一个判断指标,F1-Score的值是从0到1的,1是最好,0是最差
: 另外一个综合Precision和Recall的标准,F1-Score的变形
再举个例子:
混淆矩阵网络上有很多文章,也不用说刻意地去背去记,需要的时候百度一下你就知道,混淆矩阵实现代码:
1fromsklearn.metricsimportconfusion_matrix 2 3pipe_svc.fit(X_train,y_train) 4y_pred=pipe_svc.predict(X_test) 5confmat=confusion_matrix(y_true=y_test,y_pred=y_pred) 6print(confmat)
output: [[71 1] [ 2 40]]
1fig,ax=plt.subplots(figsize=(2.5,2.5)) 2ax.matshow(confmat,cmap=plt.cm.Blues,alpha=0.3) 3foriinrange(confmat.shape[0]): 4forjinrange(confmat.shape[1]): 5ax.text(x=j,y=i,s=confmat[i,j],va='center',ha='center') 6 7plt.xlabel('predictedlabel') 8plt.ylabel('truelabel') 9 10plt.tight_layout() 11plt.show()
6.2 相关评价指标实现
分别是准确度、recall以及F1指标的实现。
1fromsklearn.metricsimportprecision_score,recall_score,f1_score 2 3print('Precision:%.3f'%precision_score(y_true=y_test,y_pred=y_pred)) 4print('Recall:%.3f'%recall_score(y_true=y_test,y_pred=y_pred)) 5print('F1:%.3f'%f1_score(y_true=y_test,y_pred=y_pred))
Precision: 0.976 Recall: 0.952 F1: 0.964
指定评价指标自动选出最优模型:
可以通过在make_scorer中设定参数,确定需要用来评价的指标(这里用了fl_score),这个函数可以直接输出结果。
1fromsklearn.metricsimportmake_scorer 2 3scorer=make_scorer(f1_score,pos_label=0) 4 5c_gamma_range=[0.01,0.1,1.0,10.0] 6 7param_grid=[{'clf__C':c_gamma_range, 8'clf__kernel':['linear']}, 9{'clf__C':c_gamma_range, 10'clf__gamma':c_gamma_range, 11'clf__kernel':['rbf']}] 12 13gs=GridSearchCV(estimator=pipe_svc, 14param_grid=param_grid, 15scoring=scorer, 16cv=10, 17n_jobs=-1) 18gs=gs.fit(X_train,y_train) 19print(gs.best_score_) 20print(gs.best_params_)
0.982798668208 {'clf__C': 0.1, 'clf__kernel': 'linear'}
6.3 ROC曲线及其实现
如果需要理解ROC曲线,那你就需要先了解一下混淆矩阵了,具体的内容可以查看一下之前的文章,这里重点引入2个概念:
真正率(true positive rate,TPR),指的是被模型正确预测的正样本的比例:
假正率(false positive rate,FPR) ,指的是被模型错误预测的正样本的比例:
ROC曲线概念:
ROC(receiver operating characteristic)接受者操作特征,其显示的是分类器的真正率和假正率之间的关系,如下图所示:
ROC曲线有助于比较不同分类器的相对性能,其曲线下方的面积为AUC(area under curve),其面积越大则分类的性能越好,理想的分类器auc=1。
ROC曲线绘制:
对于一个特定的分类器和测试数据集,显然只能得到一个分类结果,即一组FPR和TPR结果,而要得到一个曲线,我们实际上需要一系列FPR和TPR的值。
那么如何处理?很简单,我们可以根据模型预测的概率值,并且设置不同的阈值来获得不同的预测结果。什么意思?
比如说:
5个样本,真实的target(目标标签)是y=c(1,1,0,0,1)
模型分类器将预测样本为1的概率p=c(0.5,0.6,0.55,0.4,0.7)
我们需要选定阈值才能把概率转化为类别,
如果我们选定阈值为0.1,那么5个样本被分进1的类别
如果选定0.3,结果仍然一样
如果选了0.45作为阈值,那么只有样本4被分进0
之后把所有得到的所有分类结果计算FTR,PTR,并绘制成线,就可以得到ROC曲线了,当threshold(阈值)取值越多,ROC曲线越平滑。
ROC曲线代码实现:
1fromsklearn.metricsimportroc_curve,auc 2fromscipyimportinterp 3 4pipe_lr=Pipeline([('scl',StandardScaler()), 5('pca',PCA(n_components=2)), 6('clf',LogisticRegression(penalty='l2', 7random_state=0, 8C=100.0))]) 9 10X_train2=X_train[:,[4,14]] 11 # 因为全部特征丢进去的话,预测效果太好,画ROC曲线不好看哈哈哈,所以只是取了2个特征 12 13 14cv=list(StratifiedKFold(n_splits=3, 15random_state=1).split(X_train,y_train)) 16 17fig=plt.figure(figsize=(7,5)) 18 19mean_tpr=0.0 20mean_fpr=np.linspace(0,1,100) 21all_tpr=[] 22 23fori,(train,test)inenumerate(cv): 24probas=pipe_lr.fit(X_train2[train], 25y_train[train]).predict_proba(X_train2[test]) 26 27fpr,tpr,thresholds=roc_curve(y_train[test], 28probas[:,1], 29pos_label=1) 30mean_tpr+=interp(mean_fpr,fpr,tpr) 31mean_tpr[0]=0.0 32roc_auc=auc(fpr,tpr) 33plt.plot(fpr, 34tpr, 35lw=1, 36label='ROCfold%d(area=%0.2f)' 37%(i+1,roc_auc)) 38 39plt.plot([0,1], 40[0,1], 41linestyle='--', 42color=(0.6,0.6,0.6), 43label='randomguessing') 44 45mean_tpr/=len(cv) 46mean_tpr[-1]=1.0 47mean_auc=auc(mean_fpr,mean_tpr) 48plt.plot(mean_fpr,mean_tpr,'k--', 49label='meanROC(area=%0.2f)'%mean_auc,lw=2) 50plt.plot([0,0,1], 51[0,1,1], 52lw=2, 53linestyle=':', 54color='black', 55label='perfectperformance') 56 57plt.xlim([-0.05,1.05]) 58plt.ylim([-0.05,1.05]) 59plt.xlabel('falsepositiverate') 60plt.ylabel('truepositiverate') 61plt.title('ReceiverOperatorCharacteristic') 62plt.legend(loc="lowerright") 63 64plt.tight_layout() 65plt.show()
查看下AUC和准确率的结果:
1pipe_lr=pipe_lr.fit(X_train2,y_train) 2y_labels=pipe_lr.predict(X_test[:,[4,14]]) 3y_probas=pipe_lr.predict_proba(X_test[:,[4,14]])[:,1] 4#notethatweuseprobabilitiesforroc_auc 5#the`[:,1]`selectsthepositiveclasslabelonly
1fromsklearn.metricsimportroc_auc_score,accuracy_score 2print('ROCAUC:%.3f'%roc_auc_score(y_true=y_test,y_score=y_probas)) 3print('Accuracy:%.3f'%accuracy_score(y_true=y_test,y_pred=y_labels))
ROC AUC: 0.752 Accuracy: 0.711
责任编辑:xj
原文标题:万字长文总结机器学习的模型评估与调参,附代码下载
文章出处:【微信公众号:人工智能与大数据技术】欢迎添加关注!文章转载请注明出处。
-
机器学习
+关注
关注
66文章
8460浏览量
133412 -
嵌套
+关注
关注
0文章
16浏览量
7971
原文标题:万字长文总结机器学习的模型评估与调参,附代码下载
文章出处:【微信号:TheBigData1024,微信公众号:人工智能与大数据技术】欢迎添加关注!文章转载请注明出处。
发布评论请先 登录
相关推荐
机器学习模型市场前景如何
BP神经网络的调参技巧与建议
xgboost超参数调优技巧 xgboost在图像分类中的应用
cmp在机器学习中的作用 如何使用cmp进行数据对比
LSTM神经网络的调参技巧
AI大模型与传统机器学习的区别
机器学习中的数据分割方法
Al大模型机器人
手把手教学!HLK-LD2410B/C新手必看攻略!测试教程、模块调参一文详解~
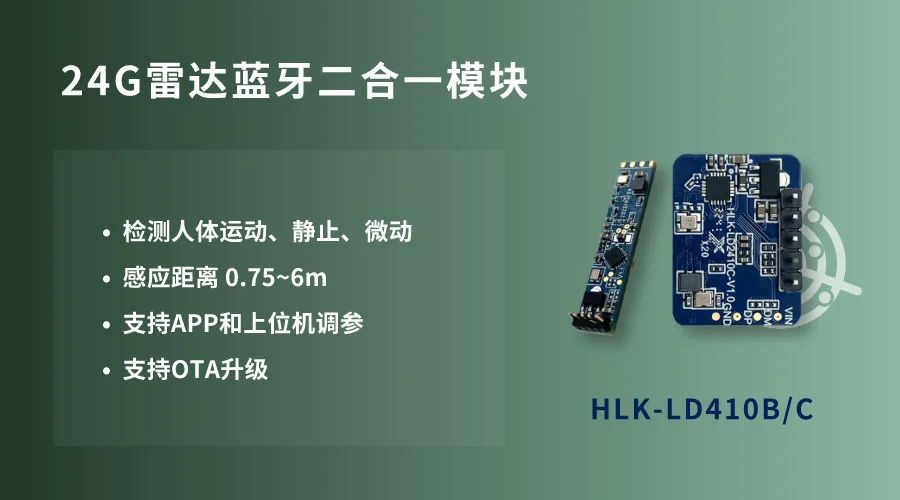
评论