在材料科学领域,制备具有特定光学性能纳米材料的方法极为重要。近年来,机器学习方法已用于解决材料科学中的众多方面,例如药物发现、医学成像、材料合成、功能分子生成和材料降解等等。然而,目前机器学习的算法大多是基于计算模拟的数据或从文献中收集的实验数据而开发的,这导致输出的目标材料难以在实验上合成,限制了其实际应用。
来自新加坡国立大学化学与生物分子工程系Xiaonan Wang教授领衔的团队,提出了一个两步式理论框架,以实现机器学习驱动的高通量微流控平台,并用于快速生产具有所需吸收光谱的银纳米颗粒。作者结合高斯过程的贝叶斯优化算法与深度神经网络,并与高通量实验合成一个循环,有效地优化了银纳米颗粒的合成。此外,此框架还训练了一种可转移算法,可以使用最终的深度神经网络进行逆设计,以合成具有不同光学特性的纳米粒子。作者所开发的方法适用于微流体高通量实验回路中其他材料的合成,同时还适用于其他类型的高通量实验平台,具有重要的应用价值。
该文近期发表于npj Computational Materials7: 55(2021),英文标题与摘要如下,点击左下角“阅读原文”可以自由获取论文PDF。
Two-step machine learning enables optimized nanoparticle synthesis
Flore Mekki-Berrada, Zekun Ren, Tan Huang, Wai Kuan Wong, Fang Zheng, Jiaxun Xie, Isaac Parker Siyu Tian, Senthilnath Jayavelu, Zackaria Mahfoud, Daniil Bash, Kedar Hippalgaonkar, Saif Khan, Tonio Buonassisi, Qianxiao Li & Xiaonan Wang
In materials science, the discovery of recipes that yield nanomaterials with defined optical properties is costly and time-consuming. In this study, we present a two-step framework for a machine learning-driven high-throughput microfluidic platform to rapidly produce silver nanoparticles with the desired absorbance spectrum. Combining a Gaussian process-based Bayesian optimization (BO) with a deep neural network (DNN), the algorithmic framework is able to converge towards the target spectrum after sampling 120 conditions. Once the dataset is large enough to train the DNN with sufficient accuracy in the region of the target spectrum, the DNN is used to predict the colour palette accessible with the reaction synthesis. While remaining interpretable by humans, the proposed framework efficiently optimizes the nanomaterial synthesis and can extract fundamental knowledge of the relationship between chemical composition and optical properties, such as the role of each reactant on the shape and amplitude of the absorbance spectrum.
文章出处:【微信公众号:知社学术圈】
责任编辑:gt
-
神经网络
+关注
关注
42文章
4789浏览量
101530 -
机器学习
+关注
关注
66文章
8459浏览量
133373
原文标题:npj: 纳米材料的精确合成—机器学习的高通量微流控平台
文章出处:【微信号:zhishexueshuquan,微信公众号:知社学术圈】欢迎添加关注!文章转载请注明出处。
发布评论请先 登录
相关推荐
《AI Agent 应用与项目实战》阅读心得2——客服机器人、AutoGen框架 、生成式代理
2025-2030年微流控行业发展趋势预测
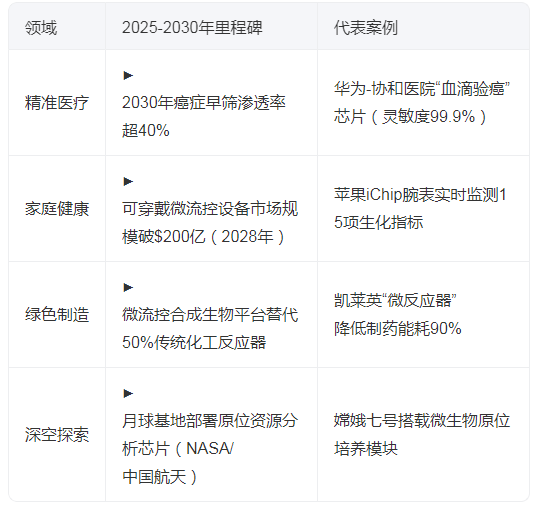
高通量玻璃微流道反应器
微流控芯片在细胞培养检测中的应用
高通量生物分析技术之微流控芯片
S型微流控芯片的优势
TensorFlow与PyTorch深度学习框架的比较与选择
中国移动浙江公司携手华为完成5G-A高通量UPF全球现网首呼
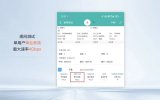
赋能产业互联网,高通量计算让世界更高效!
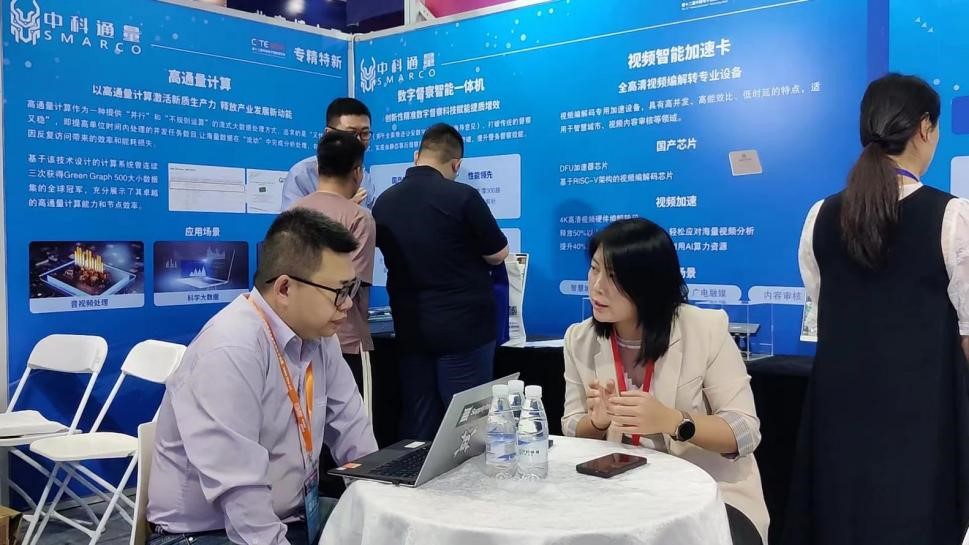
评论