从代码的角度理解YOLO V5的工作。YOLO V5的网络结构图如下:
1、与YOLO V4的区别
Yolov4在Yolov3的基础上进行了很多的创新。比如输入端采用mosaic数据增强,Backbone上采用了CSPDarknet53、Mish激活函数、Dropblock等方式,Neck中采用了SPP、FPN+PAN的结构,输出端则采用CIOU_Loss、DIOU_nms操作。因此Yolov4对Yolov3的各个部分都进行了很多的整合创新。这里给出YOLO V4的网络结构图:
Yolov5的结构其实和Yolov4的结构还是有一定的相似之处的,但也有一些不同,这里还是按照从整体到细节的方式,对每个板块进行讲解。这里给出YOLO V4的网络结构图:
通过Yolov5的网络结构图可以看到,依旧是把模型分为4个部分,分别是:输入端、Backbone、Neck、Prediction。
1.1、输入端的区别
1 Mosaic数据增强
Mosaic是参考CutMix数据增强的方式,但CutMix只使用了两张图片进行拼接,而Mosaic数据增强则采用了4张图片,随机缩放、随机裁剪、随机排布的方式进行拼接。
主要有几个优点:
1、丰富数据集:随机使用4张图片,随机缩放,再随机分布进行拼接,大大丰富了检测数据集,特别是随机缩放增加了很多小目标,让网络的鲁棒性更好。
2、减少GPU:可能会有人说,随机缩放,普通的数据增强也可以做,但作者考虑到很多人可能只有一个GPU,因此Mosaic增强训练时,可以直接计算4张图片的数据,使得Mini-batch大小并不需要很大,一个GPU就可以达到比较好的效果。
2 自适应锚框计算
在Yolov3、Yolov4中,训练不同的数据集时,计算初始锚框的值是通过单独的程序运行的。但Yolov5中将此功能嵌入到代码中,每次训练时,自适应的计算不同训练集中的最佳锚框值。
比如Yolov5在Coco数据集上初始设定的锚框:
3 自适应图片缩放
在常用的目标检测算法中,不同的图片长宽都不相同,因此常用的方式是将原始图片统一缩放到一个标准尺寸,再送入检测网络中。比如Yolo算法中常用416×416,608×608等尺寸,比如对下面800×600的图像进行变换。
但Yolov5代码中对此进行了改进,也是Yolov5推理速度能够很快的一个不错的trick。作者认为,在项目实际使用时,很多图片的长宽比不同。因此缩放填充后,两端的黑边大小都不同,而如果填充的比较多,则存在信息冗余,影响推理速度。
具体操作的步骤:
1 计算缩放比例
原始缩放尺寸是416×416,都除以原始图像的尺寸后,可以得到0.52,和0.69两个缩放系数,选择小的缩放系数0.52。
2 计算缩放后的尺寸
原始图片的长宽都乘以最小的缩放系数0.52,宽变成了416,而高变成了312。
3 计算黑边填充数值
将416-312=104,得到原本需要填充的高度。再采用numpy中np.mod取余数的方式,得到40个像素,再除以2,即得到图片高度两端需要填充的数值。
1.2、Backbone的区别
1 Focus结构
Focus结构,在Yolov3&Yolov4中并没有这个结构,其中比较关键是切片操作。比如右图的切片示意图,4×4×3的图像切片后变成3×3×12的特征图。以Yolov5s的结构为例,原始608×608×3的图像输入Focus结构,采用切片操作,先变成304×304×12的特征图,再经过一次32个卷积核的卷积操作,最终变成304×304×32的特征图。
需要注意的是:Yolov5s的Focus结构最后使用了32个卷积核,而其他三种结构,使用的数量有所增加,先注意下,后面会讲解到四种结构的不同点。
class Focus(nn.Module):
# Focus wh information into c-space
def __init__(self, c1, c2, k=1):
super(Focus, self).__init__()
self.conv = Conv(c1 * 4, c2, k, 1)
def forward(self, x): # x(b,c,w,h) -》 y(b,4c,w/2,h/2)
return self.conv(torch.cat([x[。.., ::2, ::2], x[。.., 1::2, ::2], x[。.., ::2, 1::2], x[。.., 1::2, 1::2]], 1))
2 CSP结构
Yolov5与Yolov4不同点在于,Yolov4中只有主干网络使用了CSP结构,而Yolov5中设计了两种CSP结构,以Yolov5s网络为例,以CSP1_X结构应用于Backbone主干网络,另一种CSP2_X结构则应用于Neck中。
classConv(nn.Module): #Standardconvolution def__init__(self,c1,c2,k=1,s=1,g=1,act=True):#ch_in,ch_out,kernel,stride,groups super(Conv,self).__init__() self.conv=nn.Conv2d(c1,c2,k,s,k//2,groups=g,bias=False) self.bn=nn.BatchNorm2d(c2) self.act=nn.LeakyReLU(0.1,inplace=True)ifactelsenn.Identity() defforward(self,x): returnself.act(self.bn(self.conv(x))) deffuseforward(self,x): returnself.act(self.conv(x)) classBottleneck(nn.Module): #Standardbottleneck def__init__(self,c1,c2,shortcut=True,g=1,e=0.5):#ch_in,ch_out,shortcut,groups,expansion super(Bottleneck,self).__init__() c_=int(c2*e)#hiddenchannels self.cv1=Conv(c1,c_,1,1) self.cv2=Conv(c_,c2,3,1,g=g) self.add=shortcutandc1==c2 defforward(self,x): returnx+self.cv2(self.cv1(x))ifself.addelseself.cv2(self.cv1(x)) classBottleneckCSP(nn.Module): #CSPBottleneckhttps://github.com/WongKinYiu/CrossStagePartialNetworks def__init__(self,c1,c2,n=1,shortcut=True,g=1,e=0.5):#ch_in,ch_out,number,shortcut,groups,expansion super(BottleneckCSP,self).__init__() c_=int(c2*e)#hiddenchannels self.cv1=Conv(c1,c_,1,1) self.cv2=nn.Conv2d(c1,c_,1,1,bias=False) self.cv3=nn.Conv2d(c_,c_,1,1,bias=False) self.cv4=Conv(c2,c2,1,1) self.bn=nn.BatchNorm2d(2*c_)#appliedtocat(cv2,cv3) self.act=nn.LeakyReLU(0.1,inplace=True) self.m=nn.Sequential(*[Bottleneck(c_,c_,shortcut,g,e=1.0)for_inrange(n)]) defforward(self,x): y1=self.cv3(self.m(self.cv1(x))) y2=self.cv2(x) returnself.cv4(self.act(self.bn(torch.cat((y1,y2),dim=1))))
1.3、Neck的区别
Yolov5现在的Neck和Yolov4中一样,都采用FPN+PAN的结构,但在Yolov5刚出来时,只使用了FPN结构,后面才增加了PAN结构,此外网络中其他部分也进行了调整。
Yolov5和Yolov4的不同点在于,Yolov4的Neck中,采用的都是普通的卷积操作。而Yolov5的Neck结构中,采用借鉴CSPNet设计的CSP2结构,加强网络特征融合的能力。
1.4、输出端的区别
1 Bounding box损失函数
而Yolov4中采用CIOU_Loss作为目标Bounding box的损失。而Yolov5中采用其中的GIOU_Loss做Bounding box的损失函数。
defcompute_loss(p,targets,model):#predictions,targets,model ft=torch.cuda.FloatTensorifp[0].is_cudaelsetorch.Tensor lcls,lbox,lobj=ft([0]),ft([0]),ft([0]) tcls,tbox,indices,anchors=build_targets(p,targets,model)#targets h=model.hyp#hyperparameters red='mean'#Lossreduction(sumormean) #Definecriteria BCEcls=nn.BCEWithLogitsLoss(pos_weight=ft([h['cls_pw']]),reduction=red) BCEobj=nn.BCEWithLogitsLoss(pos_weight=ft([h['obj_pw']]),reduction=red) #classlabelsmoothinghttps://arxiv.org/pdf/1902.04103.pdfeqn3 cp,cn=smooth_BCE(eps=0.0) #focalloss g=h['fl_gamma']#focallossgamma ifg>0: BCEcls,BCEobj=FocalLoss(BCEcls,g),FocalLoss(BCEobj,g) #peroutput nt=0#targets fori,piinenumerate(p):#layerindex,layerpredictions b,a,gj,gi=indices[i]#image,anchor,gridy,gridx tobj=torch.zeros_like(pi[...,0])#targetobj nb=b.shape[0]#numberoftargets ifnb: nt+=nb#cumulativetargets ps=pi[b,a,gj,gi]#predictionsubsetcorrespondingtotargets #GIoU pxy=ps[:,:2].sigmoid()*2.-0.5 pwh=(ps[:,2:4].sigmoid()*2)**2*anchors[i] pbox=torch.cat((pxy,pwh),1)#predictedbox giou=bbox_iou(pbox.t(),tbox[i],x1y1x2y2=False,GIoU=True)#giou(prediction,target) lbox+=(1.0-giou).sum()ifred=='sum'else(1.0-giou).mean()#giouloss #Obj tobj[b,a,gj,gi]=(1.0-model.gr)+model.gr*giou.detach().clamp(0).type(tobj.dtype)#giouratio #Class ifmodel.nc>1:#clsloss(onlyifmultipleclasses) t=torch.full_like(ps[:,5:],cn)#targets t[range(nb),tcls[i]]=cp lcls+=BCEcls(ps[:,5:],t)#BCE #Appendtargetstotextfile #withopen('targets.txt','a')asfile: #[file.write('%11.5g'*4%tuple(x)+' ')forxintorch.cat((txy[i],twh[i]),1)] lobj+=BCEobj(pi[...,4],tobj)#objloss lbox*=h['giou'] lobj*=h['obj'] lcls*=h['cls'] bs=tobj.shape[0]#batchsize ifred=='sum': g=3.0#lossgain lobj*=g/bs ifnt: lcls*=g/nt/model.nc lbox*=g/nt loss=lbox+lobj+lcls returnloss*bs,torch.cat((lbox,lobj,lcls,loss)).detach()
2 NMS非极大值抑制
Yolov4在DIOU_Loss的基础上采用DIOU_NMS的方式,而Yolov5中采用加权NMS的方式。可以看出,采用DIOU_NMS,下方中间箭头的黄色部分,原本被遮挡的摩托车也可以检出。
在同样的参数情况下,将NMS中IOU修改成DIOU_NMS。对于一些遮挡重叠的目标,确实会有一些改进。
2、YOLOv5社交距离项目
yolov5检测要检测的视频流中的所有人,然后再计算所有检测到的人之间的相互“距离”,和现实生活中用“m”这样的单位衡量距离不一样的是,在计算机中,简单的方法是用检测到的两个人的质心,也就是检测到的目标框的中心之间相隔的像素值作为计算机中的“距离”来衡量视频中的人之间的距离是否超过安全距离。
构建步骤:
使用目标检测算法检测视频流中的所有人,得到位置信息和质心位置;
计算所有检测到的人质心之间的相互距离;
设置安全距离,计算每个人之间的距离对,检测两个人之间的距离是否小于N个像素,小于则处于安全距离,反之则不处于。
项目架构:
detect.py代码注释如下:
importargparse fromutils.datasetsimport* fromutils.utilsimport* defdetect(save_img=False): out,source,weights,view_img,save_txt,imgsz= opt.output,opt.source,opt.weights,opt.view_img,opt.save_txt,opt.img_size webcam=source=='0'orsource.startswith('rtsp')orsource.startswith('http')orsource.endswith('.txt') #Initialize device=torch_utils.select_device(opt.device) ifos.path.exists(out): shutil.rmtree(out)#deleteoutputfolder os.makedirs(out)#makenewoutputfolder half=device.type!='cpu'#halfprecisiononlysupportedonCUDA #下载模型 google_utils.attempt_download(weights) #加载权重 model=torch.load(weights,map_location=device)['model'].float() #torch.save(torch.load(weights,map_location=device),weights)#updatemodelifSourceChangeWarning #model.fuse() #设置模型为推理模式 model.to(device).eval() ifhalf: model.half()#toFP16 #Second-stageclassifier classify=False ifclassify: modelc=torch_utils.load_classifier(name='resnet101',n=2)#initialize modelc.load_state_dict(torch.load('weights/resnet101.pt',map_location=device)['model'])#loadweights modelc.to(device).eval() #设置Dataloader vid_path,vid_writer=None,None ifwebcam: view_img=True torch.backends.cudnn.benchmark=True#setTruetospeedupconstantimagesizeinference dataset=LoadStreams(source,img_size=imgsz) else: save_img=True dataset=LoadImages(source,img_size=imgsz) #获取检测类别的标签名称 names=model.namesifhasattr(model,'names')elsemodel.modules.names #定义颜色 colors=[[random.randint(0,255)for_inrange(3)]for_inrange(len(names))] #开始推理 t0=time.time() #初始化一张全为0的图片 img=torch.zeros((1,3,imgsz,imgsz),device=device) _=model(img.half()ifhalfelseimg)ifdevice.type!='cpu'elseNone forpath,img,im0s,vid_capindataset: img=torch.from_numpy(img).to(device) img=img.half()ifhalfelseimg.float()#uint8tofp16/32 img/=255.0#0-255to0.0-1.0 ifimg.ndimension()==3: img=img.unsqueeze(0) #预测结果 t1=torch_utils.time_synchronized() pred=model(img,augment=opt.augment)[0] #使用NMS pred=non_max_suppression(pred,opt.conf_thres,opt.iou_thres,fast=True,classes=opt.classes,agnostic=opt.agnostic_nms) t2=torch_utils.time_synchronized() #进行分类 ifclassify: pred=apply_classifier(pred,modelc,img,im0s) people_coords=[] #处理预测得到的检测目标 fori,detinenumerate(pred): ifwebcam: p,s,im0=path[i],'%g:'%i,im0s[i].copy() else: p,s,im0=path,'',im0s save_path=str(Path(out)/Path(p).name) s+='%gx%g'%img.shape[2:]#printstring gn=torch.tensor(im0.shape)[[1,0,1,0]]#normalizationgainwhwh ifdetisnotNoneandlen(det): #把boxesresize到im0的size det[:,:4]=scale_coords(img.shape[2:],det[:,:4],im0.shape).round() #打印结果 forcindet[:,-1].unique(): n=(det[:,-1]==c).sum()#detectionsperclass s+='%g%ss,'%(n,names[int(c)])#addtostring #书写结果 for*xyxy,conf,clsindet: ifsave_txt: #xyxy2xywh==>把预测得到的坐标结果[x1,y1,x2,y2]转换为[x,y,w,h]其中xy1=top-left,xy2=bottom-right xywh=(xyxy2xywh(torch.tensor(xyxy).view(1,4))/gn).view(-1).tolist()#normalizedxywh withopen(save_path[:save_path.rfind('.')]+'.txt','a')asfile: file.write(('%g'*5+' ')%(cls,*xywh))#labelformat ifsave_imgorview_img:#Addbboxtoimage label='%s%.2f'%(names[int(cls)],conf) iflabelisnotNone: if(label.split())[0]=='person': #print(xyxy) people_coords.append(xyxy) #plot_one_box(xyxy,im0,line_thickness=3) plot_dots_on_people(xyxy,im0) #通过people_coords绘制people之间的连接线 #这里主要分为"LowRisk"和"HighRisk" distancing(people_coords,im0,dist_thres_lim=(200,250)) #Printtime(inference+NMS) print('%sDone.(%.3fs)'%(s,t2-t1)) #Streamresults ifview_img: cv2.imshow(p,im0) ifcv2.waitKey(1)==ord('q'):#qtoquit raiseStopIteration #Saveresults(imagewithdetections) ifsave_img: ifdataset.mode=='images': cv2.imwrite(save_path,im0) else: ifvid_path!=save_path:#newvideo vid_path=save_path ifisinstance(vid_writer,cv2.VideoWriter): vid_writer.release()#releasepreviousvideowriter fps=vid_cap.get(cv2.CAP_PROP_FPS) w=int(vid_cap.get(cv2.CAP_PROP_FRAME_WIDTH)) h=int(vid_cap.get(cv2.CAP_PROP_FRAME_HEIGHT)) vid_writer=cv2.VideoWriter(save_path,cv2.VideoWriter_fourcc(*opt.fourcc),fps,(w,h)) vid_writer.write(im0) ifsave_txtorsave_img: print('Resultssavedto%s'%os.getcwd()+os.sep+out) ifplatform=='darwin':#MacOS os.system('open'+save_path) print('Done.(%.3fs)'%(time.time()-t0)) if__name__=='__main__': parser=argparse.ArgumentParser() parser.add_argument('--weights',type=str,default='./weights/yolov5s.pt',help='model.ptpath') parser.add_argument('--source',type=str,default='./inference/videos/',help='source')#file/folder,0forwebcam parser.add_argument('--output',type=str,default='./inference/output',help='outputfolder')#outputfolder parser.add_argument('--img-size',type=int,default=640,help='inferencesize(pixels)') parser.add_argument('--conf-thres',type=float,default=0.4,help='objectconfidencethreshold') parser.add_argument('--iou-thres',type=float,default=0.5,help='IOUthresholdforNMS') parser.add_argument('--fourcc',type=str,default='mp4v',help='outputvideocodec(verifyffmpegsupport)') parser.add_argument('--device',default='0',help='cudadevice,i.e.0or0,1,2,3orcpu') parser.add_argument('--view-img',action='store_true',help='displayresults') parser.add_argument('--save-txt',action='store_true',help='saveresultsto*.txt') parser.add_argument('--classes',nargs='+',type=int,help='filterbyclass') parser.add_argument('--agnostic-nms',action='store_true',help='class-agnosticNMS') parser.add_argument('--augment',action='store_true',help='augmentedinference') opt=parser.parse_args() opt.img_size=check_img_size(opt.img_size) print(opt) withtorch.no_grad(): detect()
审核编辑:郭婷
-
gpu
+关注
关注
28文章
4742浏览量
128968 -
代码
+关注
关注
30文章
4790浏览量
68649 -
数据集
+关注
关注
4文章
1208浏览量
24712
原文标题:项目实践 | 基于YOLO-V5实现行人社交距离风险提示
文章出处:【微信号:vision263com,微信公众号:新机器视觉】欢迎添加关注!文章转载请注明出处。
发布评论请先 登录
相关推荐
助力AIoT应用:在米尔FPGA开发板上实现Tiny YOLO V4
神经网络结构搜索有什么优势?
TD-SCDMA网络结构
DeviceNet 网络结构
环形网络,环形网络结构是什么?
网络结构中链接寿命的研究
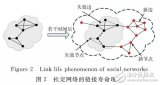
评论