Xilinx 的 Versal AI Core 系列器件旨在解决独特且最困难的 AI 推理问题,方法是使用高计算效率 ASIC 级 AI 计算引擎和灵活的可编程结构来构建具有加速器的 AI 应用,从而最大限度地提高任何给定工作负载的效率,同时提供低功耗和低延迟。
Versal AI Core 系列VCK190 评估套件采用VC1902器件,该器件在产品组合中具有最佳的 AI 性能。该套件专为需要高吞吐量 AI 推理和信号处理计算性能的设计而设计。VCK190 套件的计算能力是当前服务器级 CPU 的 100 倍,并具有多种连接选项,是从云到边缘的各种应用的理想评估和原型设计平台。
图 1:赛灵思 Versal AI 内核系列 VCK190 评估套件。(图片来源:AMD, Inc)
VCK190 评估套件的主要特性
- 板载 Versal AI 核心系列设备
- 用于前沿应用开发的最新连接技术
- 协同优化工具和调试方法
利用赛灵思 Versal AI 内核系列器件实现 AI 接口加速
图 2:赛灵思 Versal AI 内核 VC1902 ACAP 器件框图。(图片来源:AMD, Inc)
Versal® AI Core 自适应计算加速平台 (ACAP) 是一款高度集成的多核异构设备,可在硬件和软件级别动态适应各种 AI 工作负载,使其成为 AI 边缘计算应用或云加速器卡的理想选择。该平台集成了用于嵌入式计算的下一代标量引擎、用于硬件灵活性的自适应引擎,以及由 DSP 引擎和用于推理和信号处理的革命性 AI 引擎组成的智能引擎。其结果是一个适应性强的加速器,其性能、延迟和能效超过了传统 FPGA 和 GPU 的性能、延迟和能效,适用于 AI/ML 工作负载。
Versal ACAP 平台亮点
VCK190 人工智能推理性能
与当前服务器级 CPU 相比,VCK190 能够提供超过 100 倍的计算性能。下面是基于 C32B6 DPU 内核的 AI 引擎实现的性能示例,批处理 = 6。有关 VCK190 上各种神经网络样本的吞吐量性能(以帧/秒或 fps 为单位),DPU 以 1250 MHz 运行,请参阅下表。
| | No | Neural Network | Input Size | GOPS | Performance (fps) (Multiple thread) |
| ---- | -------------------------- | ------------ | ------ | ------------------------------------- |
| 1 | face_landmark | 96x72 | 0.14 | 24605.3 |
| 2 | facerec_resnet20 | 112x96 | 3.5 | 5695.3 |
| 3 | inception_v2 | 224x224 | 4 | 1845.8 |
| 4 | medical_seg_cell_tf2 | 128x128 | 5.3 | 3036.3 |
| 5 | MLPerf_resnet50_v1.5_tf | 224x224 | 8.19 | 2744.2 |
| 6 | RefineDet-Medical_EDD_tf | 320x320 | 9.8 | 1283.6 |
| 7 | tiny_yolov3_vmss | 416x416 | 5.46 | 1424.4 |
| 8 | yolov2_voc_pruned_0_77 | 448x448 | 7.8 | 1366.0 |
Table 1: Example of VCK190 AI Inference performance.
See more detail of VCK190 AI performance from Vitis AI Library User Guide (UG1354), r2.5.0 at https://docs.xilinx.com/r/en-US/ug1354-xilinx-ai-sdk/VCK190-Evaluation-Board
How Design Gateway's IP cores accelerate AI application performance?
Design Gateway's IP Cores are designed to handle Networking and Data Storage protocol without need for CPU intervention. This makes it ideal to fully offload CPU systems from complicated protocol processing and which enables them to utilize most of their computing power for AI applications including AI inference, pre and post data processing, user interface, network communication and data storage access for the best possible performance.
Figure 3: Block diagram of example an AI Application with Design Gateway's IP Cores. (Image source: Design Gateway)
Design Gateway's TCP Offload Engine IP (TOExxG-IP) performance
Processing high speed, high throughput TCP data streams over 10GbE or 25GbE by traditional CPU systems needs more than 50% of CPU time which reduces overall performance of AI applications. According to 10G TCP performance test on Xilinx's MPSoC Linux systems, CPU usage during 10GbE TCP transmission is more than 50%, TCP send and receive data transfer speed could be achieved just around 40% to 60% of 10GbE speed or 400 MB/s to 600 MB/s.
By implementing Design Gateway's TOExxG-IP Core, CPU usage for TCP transmission over 10GbE and 25GbE can be reduced to almost 0% while ethernet bandwidth utilization can be achieved close to 100%. This allows the sending and receiving of data over the TCP network directly by pure hardware logic and be fed into the Versal AI Engine with minimum CPU usage and the lowest possible latency. Figure 4 below shows the CPU usage and TCP transmission speed comparison between TOExxG-IP and MPSoC Linux systems.
Figure 4: Performance comparison of 10G/25G TCP transmission by MPSoC Linux systems and Design Gateway's TOExxG-IP Core. (Image source: Design Gateway)
Design Gateway’s TOExxG-IP for Versal devices
Figure 5: TOExxG-IP systems overview. (Image source: Design Gateway)
The TOExxG-IP core implements the TCP/IP stack (in hardwire logic) and connects with Xilinx’s EMAC Hard IP and Ethernet Subsystem module for the lower-layer hardware interface with 10G/25G/100G Ethernet speed. The user interface of the TOExxG-IP consists of a Register interface for control signals and a FIFO interface for data signals. The TOExxG-IP is designed to connect with Xilinx's Ethernet subsystem through the AXI4-ST interface. The clock frequency of the user interface depends on the Ethernet interface speed (e.g., 156.625 MHz or 322.266 MHz).
TOExxG-IP’s features
- Full TCP/IP stack implementation without need of the CPU
- Supports one session with one TOExxG-IP
- Multi-session can be implemented by using multiple TOExxG-IP instances
- Support for both Server and Client mode (Passive/Active open and close)
- Supports Jumbo frame
- Simple data interface by standard FIFO interface
- Simple control interface by single port RAM interface
FPGA resource usages on the XCVC1902-VSVA2197-2MP-ES FPGA device are shown in Table 2 below.
| | Family | Example Device | Fmax (MHz) | CLB Regs | CLB LUTs | Slice | IOB | BRAMTile^1^ | URAM | Design Tools |
| ---------------- | -------------------------- | ------------ | ---------- | ---------- | ------- | ----- | -------------- | ------ | -------------- |
| Versal AI Core | XCVC1902-VSVA2197-2MP-ES | 350 | 11340 | 10921 | 2165 | - | 51.5 | - | Vivado2021.2 |
Table 2: Example Implementation Statistics for Versal device.
More details of the TOExxG-IP are described in its datasheet which can be downloaded from Design Gateway’s website at the following links:
Design Gateway's NVMe Host Controller IP performance
NVMe Storage interface speed with PCIe Gen3 x4 or PCIe Gen4 x4 has data rates up to 32 Gbps and 64 Gbps. This is three to six times higher than 10GbE Ethernet speed. Processing complicated NVMe storage protocol by the CPU to achieve the highest possible disk access speed requires more CPU time than TCP protocol over 10GbE.
Design Gateway solved this problem by developing the NVMe IP core that is able to run as a standalone NVMe host controller, able to communicate with an NVMe SSD directly without the CPU. This enables a high efficiency and performance of the NVMe PCIe Gen3 and Gen4 SSD access, which simplifies the user interface and standard features for ease of usage without needing knowledge of the NVMe protocol. NVMe PCIe Gen4 SSD performance can achieve up to a 6 GB/s transfer speed with NVMe IP as shown in Figure 6.
Figure 6: Performance comparison of NVMe PCIe Gen3 and Gen4 SSD with Design Gateway's NVMe-IP Core. (Image source: Design Gateway)
Design Gateway's NVMe-IP’s for Versal devices
图 7:NVMe-IP 系统概述。(图片来源:设计网关)
NVMe-IP的功能
- 能够实现应用层、事务层、数据链路层和物理层的某些部分,无需CPU或外部DDR存储器即可访问NVMe SSD
- 与赛灵思 PCIe 第 3 代和第 4 代硬 IP 配合使用
- 能够利用BRAM和URAM作为数据缓冲区,而无需外部存储器接口
- 支持六个命令:识别、关机、写入、读取、SMART 和刷新(提供可选的附加命令支持)
XCVC1902-VSVA2197-2MP-E-S FPGA 器件上的 FPGA 资源使用情况如表 2 所示。
| | 家庭 | 示例设备 | 最大频率 (兆赫) | 负载均衡注册 | 负载均衡 LUT | 片 | IOB | 布拉姆蒂勒^1^ | 乌兰 | 设计工具 |
| ---------------- | -------------------------- | ------------------- | -------------- | -------------- | ------ | ----- | ---------------- | ------ | ------------ |
| Versal AI Core | XCVC1902-VSVA2197-2MP-ES | 375 | 6280 | 3948 | 1050 | - | 4 | 8 | 万岁2022.1 |
表 3:Versal 设备的实现统计信息示例。
有关 Versal 器件的 NVMe-IP 的更多详细信息,请参见其数据表,可通过以下链接从 Design Gateway 的网站下载:
结论
TOExxG-IP 和 NVMe-IP 内核都可以通过将 CPU 系统从计算和内存密集型协议(如 TCP 和 NVMe 存储协议)中完全卸载来帮助加速 AI 应用程序性能,这对于实时 AI 应用程序至关重要。这使得赛灵思的 Versal AI Core 系列器件能够执行 AI 推理和高性能计算应用,而不会出现网络和数据存储协议处理的瓶颈或延迟。
VCK190 评估套件和 Design Gateway 的网络和存储 IP 解决方案可在 Xilinx 的 Versal AI Core 器件上以尽可能低的 FPGA 资源使用量和极高的能效在 AI 应用中实现最佳性能。
-
网关
+关注
关注
9文章
4453浏览量
51084
发布评论请先 登录
相关推荐
嵌入式和人工智能究竟是什么关系?
《AI for Science:人工智能驱动科学创新》第6章人AI与能源科学读后感
AI for Science:人工智能驱动科学创新》第4章-AI与生命科学读后感
《AI for Science:人工智能驱动科学创新》第一章人工智能驱动的科学创新学习心得
risc-v在人工智能图像处理应用前景分析
人工智能ai4s试读申请
名单公布!【书籍评测活动NO.44】AI for Science:人工智能驱动科学创新
【xG24 Matter开发套件试用体验】深入了解Silicon Labs xG24 Matter开发套件
FPGA在人工智能中的应用有哪些?
【xG24 Matter开发套件试用体验】初识xG24 Matter开发套件
5G智能物联网课程之Aidlux下人工智能开发(SC171开发套件V2)
5G智能物联网课程之Aidlux下人工智能开发(SC171开发套件V1)
嵌入式人工智能的就业方向有哪些?
利用GPU加速在Orange Pi 5上跑LLMs:人工智能爱好者High翻了!
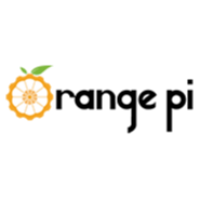
评论