特征工程是将原始数据转换为有意义的特征,以供机器学习算法使用并进行准确预测的过程。它涉及选择、提取和转换特征,以增强模型的性能。良好的特征工程可以极大地提高模型的准确性,而糟糕的特征工程则可能导致性能不佳。
Fig.1 — Feature Engineering
在本指南中,我们将介绍一系列常用的特征工程技术。我们将从特征选择和提取开始,这涉及识别数据中最重要的特征。然后,我们将转向编码分类变量,这是处理非数字数据时的重要步骤。我们还将涵盖缩放和归一化、创建新特征、处理不平衡数据、处理偏斜和峰度、处理稀有类别、处理时间序列数据、特征变换、独热编码、计数和频率编码、分箱、分组和文本预处理等内容。
通过本指南,您将全面了解特征工程技术及其如何用于提高机器学习模型的性能。让我们开始吧!
目录
1.特征选择和提取
2.编码分类变量
3.缩放和归一化
4.创建新特征
5.处理不平衡数据
6.处理偏斜和峰度
7.处理稀有类别
8.处理时间序列数据
9.文本预处理
特征选择和提取
特征选择和提取是机器学习中必不可少的部分,它涉及从数据集中选择最相关的特征,以提高模型的准确性和效率。在这里,我们将讨论一些流行的特征选择和提取方法,并提供 Python 代码片段。
** 1.主成分分析(PCA)** :PCA 是一种降维技术,通过找到一个能够捕获数据中最大方差的新特征集,从而减少数据集中的特征数量。新特征称为主成分,它们彼此正交并可用于重构原始数据集。
让我们看看如何使用 scikit-learn 对数据集执行 PCA:
from sklearn.decomposition import PCA
# create a PCA object
pca = PCA(n_components=2)
# fit and transform the data
X_pca = pca.fit_transform(X)
# calculate the explained variance ratio
print("Explained variance ratio:", pca.explained_variance_ratio_)
在这里,我们创建一个 PCA 对象并指定要提取的主成分数量。然后,我们拟合和转换数据以获得新的特征集。最后,我们计算解释的方差比率以确定每个主成分捕获了多少数据中的方差。
** 2.线性判别分析(LDA)** :LDA 是一种用于分类问题中的特征提取的监督学习技术。它通过找到一个新的特征集,最大化数据中类别之间的分离程度。
让我们看看如何使用 scikit-learn 在数据集上执行 LDA:
from sklearn.discriminant_analysis import LinearDiscriminantAnalysis
# create an LDA object
lda = LinearDiscriminantAnalysis(n_components=1)
# fit and transform the data
X_lda = lda.fit_transform(X, y)
在这里,我们创建一个 LDA 对象并指定要提取的主成分数量。然后,我们拟合和转换数据以获得新的特征集。
3.相关分析 :相关分析用于识别数据集中特征之间的相关性。高度相关的特征可以从数据集中删除,因为它们提供了冗余信息。
让我们看看如何使用 pandas 在数据集上执行相关分析:
import pandas as pd
# calculate the correlation matrix
corr_matrix = df.corr()
# select highly correlated features
high_corr = corr_matrix[abs(corr_matrix) > 0.8]
# drop highly correlated features
df = df.drop(high_corr.columns, axis=1)
在这里,我们使用 pandas 计算相关矩阵并选择高度相关的特征。然后,我们使用 drop 方法从数据集中删除高度相关的特征。
Fig.2 — Feature Selection Measures
** 4. 递归特征消除(RFE)** :RFE 是一种通过逐步考虑越来越小的特征子集来选择特征的方法。在每次迭代中,模型使用剩余的特征进行训练,并对每个特征的重要性进行排名。然后消除最不重要的特征,并重复该过程,直到获得所需数量的特征为止。
以下是使用 RFE 进行特征选择的示例:
from sklearn.feature_selection import RFE
from sklearn.linear_model import LinearRegression
from sklearn.datasets import load_boston
data = load_boston()
X, y = data.data, data.target
model = LinearRegression()
rfe = RFE(model, n_features_to_select=5)
rfe.fit(X, y)
selected_features = data.feature_names[rfe.support_]
print(selected_features)
** 5.基于树的方法** :决策树和随机森林是用于这个目的的流行的基于树的方法。在这些方法中,基于最重要的特征来预测目标变量创建了一个树结构。每个特征的重要性是通过基于该特征拆分数据导致的不纯度减少来计算的。
在决策树中,选择信息增益最高的特征作为根节点,并基于该特征拆分数据。这个过程递归重复,直到满足停止标准,例如最大树深度或每个叶子节点的最小样本数。
在随机森林中,使用特征和数据的随机子集来建立多个决策树。每个特征的重要性是通过在所有树中平均减少不纯度来计算的。这有助于降低模型的方差并提高其可推广性。
from sklearn.ensemble import RandomForestRegressor
# Load the data
X, y = load_data()
# Create a random forest regressor
rf = RandomForestRegressor(n_estimators=100, random_state=42)
# Fit the model
rf.fit(X, y)
# Get feature importances
importances = rf.feature_importances_
# Print feature importances
for feature, importance in zip(X.columns, importances):
print(feature, importance)
基于树的方法也可以用于特征提取。在这种情况下,我们可以基于树的决策边界提取新的特征。例如,我们可以使用决策树的叶节点作为新的二元特征,指示数据点是否落在特征空间的该区域内。
** 6.包装方法** :这是一种特征选择方法,其中模型在不同的特征子集上进行训练和评估。对于每个特征子集,模型的性能进行测量,并选择基于模型性能的最佳特征子集。
下面是一个使用递归特征消除(RFE)和支持向量机(SVM)分类器在 scikit-learn 中实现包装方法的示例:
from sklearn.svm import SVC
from sklearn.feature_selection import RFE
from sklearn.datasets import load_iris
# load the iris dataset
data = load_iris()
X = data.data
y = data.target
# create an SVM classifier
svm = SVC(kernel='linear')
# create a feature selector using RFE with SVM
selector = RFE(svm, n_features_to_select=2)
# fit the selector to the data
selector.fit(X, y)
# print the selected features
print(selector.support_)
print(selector.ranking_)
在这个例子中,我们首先加载鸢尾花数据集,并将其分为特征(X)和目标(y)。然后我们使用线性核创建一个 SVM 分类器。然后,我们使用 RFE 和 SVM 创建一个特征选择器,并将其拟合到数据。最后,我们使用选择器的 support_ 和 ranking_ 属性打印所选特征。
** 前向选择:** 前向选择是一种包装方法,它涉及迭代地将一个特征添加到模型中,直到模型的性能停止提高。以下是它在 Python 中的工作方式:
from sklearn.feature_selection import SequentialFeatureSelector
from sklearn.linear_model import LinearRegression
# Load the dataset
X, y = load_dataset()
# Initialize the feature selector
selector = SequentialFeatureSelector(LinearRegression(), n_features_to_select=5, direction='forward')
# Fit the feature selector
selector.fit(X, y)
# Print the selected features
print(selector.support_)
在上面的代码中,我们首先加载数据集,然后使用线性回归模型和一个指定要选择的特征数量的参数 n_features_to_select 来初始化 SequentialFeatureSelector 对象。然后,我们在数据集上拟合选择器并打印所选特征。
后向消除: 后向消除是一种包装方法,它涉及迭代地将一个特征从模型中逐步删除,直到模型的性能停止提高。以下是它在 Python 中的工作方式:
from sklearn.feature_selection import SequentialFeatureSelector
from sklearn.linear_model import LinearRegression
# Load the dataset
X, y = load_dataset()
# Initialize the feature selector
selector = SequentialFeatureSelector(LinearRegression(), n_features_to_select=5, direction='backward')
# Fit the feature selector
selector.fit(X, y)
# Print the selected features
print(selector.support_)
在上面的代码中,我们使用线性回归模型和一个参数 direction='backward' 来初始化 SequentialFeatureSelector 对象,以执行后向消除。然后,我们在数据集上拟合选择器并打印所选特征。
穷尽搜索: 穷尽搜索是一种过滤方法,它涉及评估所有可能的特征子集,并根据评分标准选择最佳的特征子集。以下是它在 Python 中的工作方式:
from itertools import combinations
from sklearn.metrics import r2_score
from sklearn.linear_model import LinearRegression
# Load the dataset
X, y = load_dataset()
# Initialize variables
best_score = -float('inf')
best_features = None
# Loop over all possible subsets of features
for k in range(1, len(X.columns) + 1):
for subset in combinations(X.columns, k):
# Train a linear regression model
X_subset = X[list(subset)]
model = LinearRegression().fit(X_subset, y)
# Compute the R2 score
score = r2_score(y, model.predict(X_subset))
# Update the best subset of features
if score > best_score:
best_score = score
best_features = subset
# Print the best subset of features
print(best_features)
在上面的代码中,我们首先加载数据集,然后使用 itertools.combinations 函数循环遍历所有可能的特征子集。对于每个子集,我们训练一个线性回归模型并计算 R2 分数。然后,根据最高的 R2 分数更新最佳特征子集,并打印所选特征。
** 7.嵌入方法** :这些方法涉及在模型训练过程中选择特征。例如,Lasso 回归和 Ridge 回归会向损失函数添加惩罚项以鼓励稀疏特征选择。
Lasso 回归 : Lasso 回归也会向损失函数添加惩罚项,但它使用的是模型系数的绝对值而不是平方。这导致了一种更加激进的特征选择过程,因为一些系数可以被设置为精确的零。Lasso 回归在处理高维数据时特别有用,因为它可以有效地减少模型使用的特征数量。
from sklearn.linear_model import Lasso
from sklearn.datasets import load_boston
from sklearn.preprocessing import StandardScaler
data = load_boston()
X = data.data
y = data.target
# Standardize the features
scaler = StandardScaler()
X = scaler.fit_transform(X)
# Fit the Lasso model
lasso = Lasso(alpha=0.1)
lasso.fit(X, y)
# Get the coefficients
coefficients = lasso.coef_
Ridge 回归:Ridge 回归向损失函数添加惩罚项,这鼓励模型选择一组更重要的特征来预测目标变量。惩罚项与模型系数的大小的平方成正比,因此它倾向于将系数缩小到零,而不是将它们精确地设置为零。
from sklearn.linear_model import Ridge
from sklearn.datasets import load_boston
from sklearn.preprocessing import StandardScaler
data = load_boston()
X = data.data
y = data.target
# Standardize the features
scaler = StandardScaler()
X = scaler.fit_transform(X)
# Fit the Ridge model
ridge = Ridge(alpha=0.1)
ridge.fit(X, y)
# Get the coefficients
coefficients = ridge.coef_
在这两种情况下,正则化参数 alpha 控制惩罚项的强度。alpha 值越高,特征选择越稀疏。
编码分类变量
编码分类变量是特征工程中的一个关键步骤,它涉及将分类变量转换为机器学习算法可以理解的数字形式。以下是用于编码分类变量的一些常见技术:
1.独热编码:
独热编码是一种将分类变量转换为一组二进制特征的技术,其中每个特征对应于原始变量中的一个唯一类别。在这种技术中,为每个类别创建一个新的二进制列,如果存在该类别,则将值设置为1,否则设置为0。
以下是使用 pandas 库的示例:
import pandas as pd
# create a sample dataframe
df = pd.DataFrame({
'color': ['red', 'blue', 'green', 'red', 'yellow', 'blue']
})
# apply one-hot encoding
one_hot_encoded = pd.get_dummies(df['color'])
print(one_hot_encoded)
2.标签编码:
标签编码是一种将原始变量中的每个类别分配一个唯一数字值的技术。在这种技术中,每个类别被赋予一个数字标签,其中标签的分配基于变量中类别的顺序。
以下是使用 scikit-learn 库的示例:
from sklearn.preprocessing import LabelEncoder
# create a sample dataframe
df = pd.DataFrame({
'color': ['red', 'blue', 'green', 'red', 'yellow', 'blue']
})
# apply label encoding
label_encoder = LabelEncoder()
df['color_encoded'] = label_encoder.fit_transform(df['color'])
print(df)
Fig.3 — Encoding Data
3.序数编码:
序数编码是一种根据类别的顺序或排名为原始变量中的每个类别分配一个数字值的技术。在这种技术中,类别根据特定标准排序,然后根据它们在排序中的位置分配数字值。
以下是使用 category_encoders 库的示例:
import category_encoders as ce
# create a sample dataframe
df = pd.DataFrame({
'size': ['S', 'M', 'L', 'XL', 'M', 'S']
})
# apply ordinal encoding
ordinal_encoder = ce.OrdinalEncoder(cols=['size'], order=['S', 'M', 'L', 'XL'])
df = ordinal_encoder.fit_transform(df)
print(df)
-
编码
+关注
关注
6文章
942浏览量
54822 -
机器学习
+关注
关注
66文章
8414浏览量
132606 -
预处理
+关注
关注
0文章
33浏览量
10478
发布评论请先 登录
相关推荐
有感FOC算法学习与实现总结
SVPWM的原理与算法学习课件免费下载
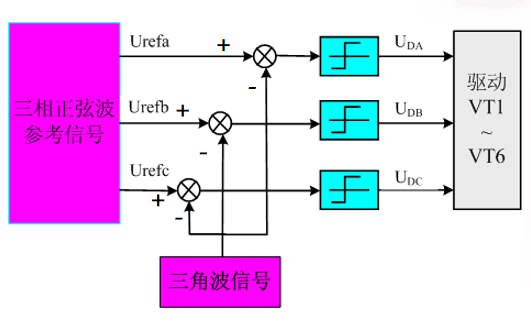
评论