虽然 AlexNet 提供了深度 CNN 可以取得良好结果的经验证据,但它没有提供通用模板来指导后续研究人员设计新网络。在接下来的部分中,我们将介绍几个常用于设计深度网络的启发式概念。
该领域的进展反映了芯片设计中 VLSI(超大规模集成)的进展,工程师从将晶体管放置到逻辑元件再到逻辑块(Mead,1980 年)。同样,神经网络架构的设计也变得越来越抽象,研究人员从单个神经元的角度思考到整个层,现在转向块,重复层的模式。十年后,这已经发展到研究人员使用整个训练模型将它们重新用于不同但相关的任务。此类大型预训练模型通常称为 基础模型 (Bommasani等人,2021 年)。
回到网络设计。使用块的想法首先出现于牛津大学的视觉几何组 (VGG),在他们同名的VGG网络中(Simonyan 和 Zisserman,2014 年)。通过使用循环和子例程,可以使用任何现代深度学习框架轻松地在代码中实现这些重复结构。
import torch from torch import nn from d2l import torch as d2l
from mxnet import init, np, npx from mxnet.gluon import nn from d2l import mxnet as d2l npx.set_np()
import jax from flax import linen as nn from d2l import jax as d2l
import tensorflow as tf from d2l import tensorflow as d2l
8.2.1. VGG 块
CNN 的基本构建块是以下序列:(i) 带有填充的卷积层以保持分辨率,(ii) 非线性,例如 ReLU,(iii) 池化层,例如最大池化以减少解决。这种方法的问题之一是空间分辨率下降得非常快。特别是,这强加了一个硬限制log2d网络上所有维度之前的卷积层(d) 用完了。例如,在 ImageNet 的情况下,以这种方式不可能有超过 8 个卷积层。
Simonyan 和 Zisserman ( 2014 )的关键思想是以 块的形式通过最大池化在下采样之间使用多个卷积。他们主要感兴趣的是深度网络还是宽网络表现更好。例如,连续应用两个 3×3卷积接触与单个相同的像素 5×5卷积确实如此。同时,后者使用了大约同样多的参数(25⋅c2) 三个 3×3卷积做(3⋅9⋅c2). 在相当详细的分析中,他们表明深度和狭窄的网络明显优于浅层网络。这将深度学习置于对具有超过 100 层的典型应用的更深网络的追求上。堆叠3×3卷积已成为后来的深度网络的黄金标准(最近Liu等人( 2022 )才重新考虑的设计决策)。因此,小卷积的快速实现已成为 GPU 的主要内容 (Lavin 和 Gray,2016 年)。
回到 VGG:一个 VGG 块由一系列卷积组成 3×3填充为 1 的内核(保持高度和宽度)后跟一个2×2步长为 2 的最大池化层(每个块后将高度和宽度减半)。在下面的代码中,我们定义了一个函数vgg_block来实现一个 VGG 块。
下面的函数有两个参数,对应于卷积层数num_convs和输出通道数 num_channels。
def vgg_block(num_convs, out_channels): layers = [] for _ in range(num_convs): layers.append(nn.LazyConv2d(out_channels, kernel_size=3, padding=1)) layers.append(nn.ReLU()) layers.append(nn.MaxPool2d(kernel_size=2,stride=2)) return nn.Sequential(*layers)
def vgg_block(num_convs, num_channels): blk = nn.Sequential() for _ in range(num_convs): blk.add(nn.Conv2D(num_channels, kernel_size=3, padding=1, activation='relu')) blk.add(nn.MaxPool2D(pool_size=2, strides=2)) return blk
def vgg_block(num_convs, out_channels): layers = [] for _ in range(num_convs): layers.append(nn.Conv(out_channels, kernel_size=(3, 3), padding=(1, 1))) layers.append(nn.relu) layers.append(lambda x: nn.max_pool(x, window_shape=(2, 2), strides=(2, 2))) return nn.Sequential(layers)
def vgg_block(num_convs, num_channels): blk = tf.keras.models.Sequential() for _ in range(num_convs): blk.add( tf.keras.layers.Conv2D(num_channels, kernel_size=3, padding='same', activation='relu')) blk.add(tf.keras.layers.MaxPool2D(pool_size=2, strides=2)) return blk
8.2.2. VGG网络
与 AlexNet 和 LeNet 一样,VGG 网络可以分为两部分:第一部分主要由卷积层和池化层组成,第二部分由与 AlexNet 相同的全连接层组成。关键区别在于卷积层在保持维数不变的非线性变换中分组,然后是分辨率降低步骤,如图 8.2.1所示。
图 8.2.1从 AlexNet 到 VGG。关键区别在于 VGG 由层块组成,而 AlexNet 的层都是单独设计的。
网络的卷积部分连续连接 图 8.2.1中的几个 VGG 块(也在vgg_block函数中定义)。这种卷积分组是一种在过去十年中几乎保持不变的模式,尽管操作的具体选择已经发生了相当大的修改。该变量 conv_arch由一个元组列表(每个块一个)组成,其中每个元组包含两个值:卷积层数和输出通道数,它们正是调用函数所需的参数vgg_block。因此,VGG 定义了一个网络家族,而不仅仅是一个特定的表现形式。要构建一个特定的网络,我们只需迭代arch以组成块。
class VGG(d2l.Classifier): def __init__(self, arch, lr=0.1, num_classes=10): super().__init__() self.save_hyperparameters() conv_blks = [] for (num_convs, out_channels) in arch: conv_blks.append(vgg_block(num_convs, out_channels)) self.net = nn.Sequential( *conv_blks, nn.Flatten(), nn.LazyLinear(4096), nn.ReLU(), nn.Dropout(0.5), nn.LazyLinear(4096), nn.ReLU(), nn.Dropout(0.5), nn.LazyLinear(num_classes)) self.net.apply(d2l.init_cnn)
class VGG(d2l.Classifier): def __init__(self, arch, lr=0.1, num_classes=10): super().__init__() self.save_hyperparameters() self.net = nn.Sequential() for (num_convs, num_channels) in arch: self.net.add(vgg_block(num_convs, num_channels)) self.net.add(nn.Dense(4096, activation='relu'), nn.Dropout(0.5), nn.Dense(4096, activation='relu'), nn.Dropout(0.5), nn.Dense(num_classes)) self.net.initialize(init.Xavier())
class VGG(d2l.Classifier): arch: list lr: float = 0.1 num_classes: int = 10 training: bool = True def setup(self): conv_blks = [] for (num_convs, out_channels) in self.arch: conv_blks.append(vgg_block(num_convs, out_channels)) self.net = nn.Sequential([ *conv_blks, lambda x: x.reshape((x.shape[0], -1)), # flatten nn.Dense(4096), nn.relu, nn.Dropout(0.5, deterministic=not self.training), nn.Dense(4096), nn.relu, nn.Dropout(0.5, deterministic=not self.training), nn.Dense(self.num_classes)])
class VGG(d2l.Classifier): def __init__(self, arch, lr=0.1, num_classes=10): super().__init__() self.save_hyperparameters() self.net = tf.keras.models.Sequential() for (num_convs, num_channels) in arch: self.net.add(vgg_block(num_convs, num_channels)) self.net.add( tf.keras.models.Sequential([ tf.keras.layers.Flatten(), tf.keras.layers.Dense(4096, activation='relu'), tf.keras.layers.Dropout(0.5), tf.keras.layers.Dense(4096, activation='relu'), tf.keras.layers.Dropout(0.5), tf.keras.layers.Dense(num_classes)]))
原始VGG网络有5个卷积块,其中前两个各有一个卷积层,后三个各有两个卷积层。第一个块有 64 个输出通道,随后的每个块将输出通道的数量加倍,直到该数量达到 512。由于该网络使用 8 个卷积层和 3 个全连接层,因此通常称为 VGG-11。
VGG(arch=((1, 64), (1, 128), (2, 256), (2, 512), (2, 512))).layer_summary( (1, 1, 224, 224))
Sequential output shape: torch.Size([1, 64, 112, 112]) Sequential output shape: torch.Size([1, 128, 56, 56]) Sequential output shape: torch.Size([1, 256, 28, 28]) Sequential output shape: torch.Size([1, 512, 14, 14]) Sequential output shape: torch.Size([1, 512, 7, 7]) Flatten output shape: torch.Size([1, 25088]) Linear output shape: torch.Size([1, 4096]) ReLU output shape: torch.Size([1, 4096]) Dropout output shape: torch.Size([1, 4096]) Linear output shape: torch.Size([1, 4096]) ReLU output shape: torch.Size([1, 4096]) Dropout output shape: torch.Size([1, 4096]) Linear output shape: torch.Size([1, 10])
VGG(arch=((1, 64), (1, 128), (2, 256), (2, 512), (2, 512))).layer_summary( (1, 1, 224, 224))
Sequential output shape: (1, 64, 112, 112) Sequential output shape: (1, 128, 56, 56) Sequential output shape: (1, 256, 28, 28) Sequential output shape: (1, 512, 14, 14) Sequential output shape: (1, 512, 7, 7) Dense output shape: (1, 4096) Dropout output shape: (1, 4096) Dense output shape: (1, 4096) Dropout output shape: (1, 4096) Dense output shape: (1, 10)
VGG(arch=((1, 64), (1, 128), (2, 256), (2, 512), (2, 512)), training=False).layer_summary((1, 224, 224, 1))
Sequential output shape: (1, 112, 112, 64) Sequential output shape: (1, 56, 56, 128) Sequential output shape: (1, 28, 28, 256) Sequential output shape: (1, 14, 14, 512) Sequential output shape: (1, 7, 7, 512) function output shape: (1, 25088) Dense output shape: (1, 4096) custom_jvp output shape: (1, 4096) Dropout output shape: (1, 4096) Dense output shape: (1, 4096) custom_jvp output shape: (1, 4096) Dropout output shape: (1, 4096) Dense output shape: (1, 10)
VGG(arch=((1, 64), (1, 128), (2, 256), (2, 512), (2, 512))).layer_summary( (1, 224, 224, 1))
Sequential output shape: (1, 112, 112, 64) Sequential output shape: (1, 56, 56, 128) Sequential output shape: (1, 28, 28, 256) Sequential output shape: (1, 14, 14, 512) Sequential output shape: (1, 7, 7, 512) Sequential output shape: (1, 10)
如您所见,我们将每个块的高度和宽度减半,最终达到 7 的高度和宽度,然后展平表示以供网络的完全连接部分处理。 Simonyan 和 Zisserman ( 2014 )描述了 VGG 的其他几种变体。事实上,在引入新架构时,提出具有不同速度-精度权衡的网络系列已经成为常态。
8.2.3. 训练
由于 VGG-11 在计算上比 AlexNet 要求更高,我们构建了一个通道数较少的网络。这对于 Fashion-MNIST 的训练来说绰绰有余。模型训练过程与8.1节AlexNet类似。再次观察验证和训练损失之间的密切匹配,表明只有少量过度拟合。
model = VGG(arch=((1, 16), (1, 32), (2, 64), (2, 128), (2, 128)), lr=0.01) trainer = d2l.Trainer(max_epochs=10, num_gpus=1) data = d2l.FashionMNIST(batch_size=128, resize=(224, 224)) model.apply_init([next(iter(data.get_dataloader(True)))[0]], d2l.init_cnn) trainer.fit(model, data)
model = VGG(arch=((1, 16), (1, 32), (2, 64), (2, 128), (2, 128)), lr=0.01) trainer = d2l.Trainer(max_epochs=10, num_gpus=1) data = d2l.FashionMNIST(batch_size=128, resize=(224, 224)) trainer.fit(model, data)
model = VGG(arch=((1, 16), (1, 32), (2, 64), (2, 128), (2, 128)), lr=0.01) trainer = d2l.Trainer(max_epochs=10, num_gpus=1) data = d2l.FashionMNIST(batch_size=128, resize=(224, 224)) trainer.fit(model, data)
trainer = d2l.Trainer(max_epochs=10) data = d2l.FashionMNIST(batch_size=128, resize=(224, 224)) with d2l.try_gpu(): model = VGG(arch=((1, 16), (1, 32), (2, 64), (2, 128), (2, 128)), lr=0.01) trainer.fit(model, data)
8.2.4. 概括
有人可能会争辩说 VGG 是第一个真正现代的卷积神经网络。虽然 AlexNet 引入了许多使深度学习大规模有效的组件,但可以说是 VGG 引入了关键属性,例如多个卷积块以及对深度和窄网络的偏好。它也是第一个实际上是整个类似参数化模型系列的网络,为从业者提供了复杂性和速度之间的充分权衡。这也是现代深度学习框架大放异彩的地方。不再需要生成 XML 配置文件来指定网络,而是通过简单的 Python 代码组装所述网络。
最近 ParNet (Goyal等人,2021 年) 证明,可以通过大量并行计算使用更浅的架构来实现有竞争力的性能。这是一个令人兴奋的发展,希望它能影响未来的建筑设计。不过,在本章的剩余部分,我们将追溯过去十年的科学进步之路。
8.2.5. 练习
与 AlexNet 相比,VGG 在计算方面要慢得多,而且需要更多的 GPU 内存。
比较 AlexNet 和 VGG 所需的参数数量。
比较卷积层和全连接层中使用的浮点运算数量。
您如何减少全连接层产生的计算成本?
当显示与网络各层相关的维度时,我们只能看到与 8 个块(加上一些辅助变换)相关的信息,即使网络有 11 层。剩下的 3 层去了哪里?
使用 VGG 论文(Simonyan 和 Zisserman,2014 年)中的表 1构建其他常见模型,例如 VGG-16 或 VGG-19。
对 Fashion-MNIST 中的分辨率进行上采样8 从28×28到224×224尺寸非常浪费。尝试修改网络架构和分辨率转换,例如,将其输入改为 56 或 84 维。你能在不降低网络准确性的情况下这样做吗?考虑 VGG 论文(Simonyan 和 Zisserman,2014 年),了解在下采样之前添加更多非线性的想法。
-
网络
+关注
关注
14文章
7571浏览量
88900 -
pytorch
+关注
关注
2文章
808浏览量
13240
发布评论请先 登录
相关推荐
基于PyTorch的深度学习入门教程之使用PyTorch构建一个神经网络
【周易AIPU 仿真】在R329上部署VGG_16网络模型
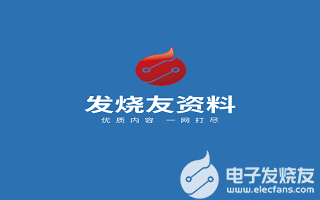
PyTorch教程8.4之多分支网络(GoogLeNet)
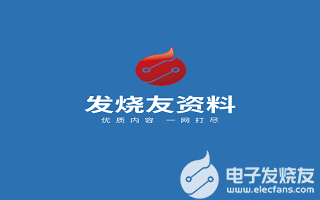
PyTorch教程8.7之密集连接网络(DenseNet)
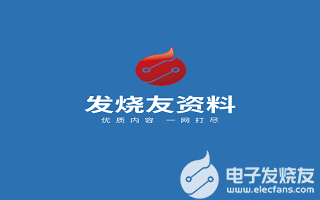
评论