当我们在10.7 节遇到机器翻译时,我们设计了一个基于两个 RNN 的序列到序列 (seq2seq) 学习的编码器-解码器架构 ( Sutskever et al. , 2014 )。具体来说,RNN 编码器将可变长度序列转换为固定形状的上下文变量。然后,RNN 解码器根据生成的标记和上下文变量逐个标记地生成输出(目标)序列标记。
回想一下我们在下面重印的图 10.7.2 (图 11.4.1)以及一些额外的细节。通常,在 RNN 中,有关源序列的所有相关信息都由编码器转换为某种内部固定维状态表示。正是这种状态被解码器用作生成翻译序列的完整和唯一的信息源。换句话说,seq2seq 机制将中间状态视为可能作为输入的任何字符串的充分统计。
图 11.4.1序列到序列模型。编码器生成的状态是编码器和解码器之间唯一共享的信息。
虽然这对于短序列来说是相当合理的,但很明显这对于长序列来说是不可行的,比如一本书的章节,甚至只是一个很长的句子。毕竟,一段时间后,中间表示中将根本没有足够的“空间”来存储源序列中所有重要的内容。因此,解码器将无法翻译又长又复杂的句子。第一个遇到的人是 格雷夫斯 ( 2013 )当他们试图设计一个 RNN 来生成手写文本时。由于源文本具有任意长度,他们设计了一个可区分的注意力模型来将文本字符与更长的笔迹对齐,其中对齐仅在一个方向上移动。这反过来又利用了语音识别中的解码算法,例如隐马尔可夫模型 (Rabiner 和 Juang,1993 年)。
受到学习对齐的想法的启发, Bahdanau等人。( 2014 )提出了一种没有单向对齐限制的可区分注意力模型。在预测标记时,如果并非所有输入标记都相关,则模型仅对齐(或关注)输入序列中被认为与当前预测相关的部分。然后,这用于在生成下一个令牌之前更新当前状态。虽然在其描述中相当无伤大雅,但这种Bahdanau 注意力机制可以说已经成为过去十年深度学习中最有影响力的想法之一,并催生了 Transformers (Vaswani等人,2017 年)以及许多相关的新架构。
import torch from torch import nn from d2l import torch as d2l
from mxnet import init, np, npx from mxnet.gluon import nn, rnn from d2l import mxnet as d2l npx.set_np()
import jax from flax import linen as nn from jax import numpy as jnp from d2l import jax as d2l
import tensorflow as tf from d2l import tensorflow as d2l
11.4.1。模型
我们遵循第 10.7 节的 seq2seq 架构引入的符号 ,特别是(10.7.3)。关键思想是,而不是保持状态,即上下文变量c将源句子总结为固定的,我们动态更新它,作为原始文本(编码器隐藏状态)的函数ht) 和已经生成的文本(解码器隐藏状态st′−1). 这产生 ct′, 在任何解码时间步后更新 t′. 假设输入序列的长度T. 在这种情况下,上下文变量是注意力池的输出:
(11.4.1)ct′=∑t=1Tα(st′−1,ht)ht.
我们用了st′−1作为查询,和 ht作为键和值。注意 ct′然后用于生成状态 st′并生成一个新令牌(参见 (10.7.3))。特别是注意力权重 α使用由 ( 11.3.7 )定义的附加注意评分函数按照 (11.3.3)计算。这种使用注意力的 RNN 编码器-解码器架构如图 11.4.2所示。请注意,后来对该模型进行了修改,例如在解码器中包含已经生成的标记作为进一步的上下文(即,注意力总和确实停止在T而是它继续进行t′−1). 例如,参见Chan等人。( 2015 )描述了这种应用于语音识别的策略。
图 11.4.2具有 Bahdanau 注意机制的 RNN 编码器-解码器模型中的层。
11.4.2。用注意力定义解码器
要实现带有注意力的 RNN 编码器-解码器,我们只需要重新定义解码器(从注意力函数中省略生成的符号可以简化设计)。让我们通过定义一个意料之中的命名类来开始具有注意力的解码器的基本接口 AttentionDecoder。
class AttentionDecoder(d2l.Decoder): #@save """The base attention-based decoder interface.""" def __init__(self): super().__init__() @property def attention_weights(self): raise NotImplementedError
class AttentionDecoder(d2l.Decoder): #@save """The base attention-based decoder interface.""" def __init__(self): super().__init__() @property def attention_weights(self): raise NotImplementedError
class AttentionDecoder(d2l.Decoder): #@save """The base attention-based decoder interface.""" def __init__(self): super().__init__() @property def attention_weights(self): raise NotImplementedError
我们需要在Seq2SeqAttentionDecoder 类中实现 RNN 解码器。解码器的状态初始化为(i)编码器最后一层在所有时间步的隐藏状态,用作注意力的键和值;(ii) 编码器在最后一步的所有层的隐藏状态。这用于初始化解码器的隐藏状态;(iii) 编码器的有效长度,以排除注意力池中的填充标记。在每个解码时间步,解码器最后一层的隐藏状态,在前一个时间步获得,用作注意机制的查询。注意机制的输出和输入嵌入都被连接起来作为 RNN 解码器的输入。
class Seq2SeqAttentionDecoder(AttentionDecoder): def __init__(self, vocab_size, embed_size, num_hiddens, num_layers, dropout=0): super().__init__() self.attention = d2l.AdditiveAttention(num_hiddens, dropout) self.embedding = nn.Embedding(vocab_size, embed_size) self.rnn = nn.GRU( embed_size + num_hiddens, num_hiddens, num_layers, dropout=dropout) self.dense = nn.LazyLinear(vocab_size) self.apply(d2l.init_seq2seq) def init_state(self, enc_outputs, enc_valid_lens): # Shape of outputs: (num_steps, batch_size, num_hiddens). # Shape of hidden_state: (num_layers, batch_size, num_hiddens) outputs, hidden_state = enc_outputs return (outputs.permute(1, 0, 2), hidden_state, enc_valid_lens) def forward(self, X, state): # Shape of enc_outputs: (batch_size, num_steps, num_hiddens). # Shape of hidden_state: (num_layers, batch_size, num_hiddens) enc_outputs, hidden_state, enc_valid_lens = state # Shape of the output X: (num_steps, batch_size, embed_size) X = self.embedding(X).permute(1, 0, 2) outputs, self._attention_weights = [], [] for x in X: # Shape of query: (batch_size, 1, num_hiddens) query = torch.unsqueeze(hidden_state[-1], dim=1) # Shape of context: (batch_size, 1, num_hiddens) context = self.attention( query, enc_outputs, enc_outputs, enc_valid_lens) # Concatenate on the feature dimension x = torch.cat((context, torch.unsqueeze(x, dim=1)), dim=-1) # Reshape x as (1, batch_size, embed_size + num_hiddens) out, hidden_state = self.rnn(x.permute(1, 0, 2), hidden_state) outputs.append(out) self._attention_weights.append(self.attention.attention_weights) # After fully connected layer transformation, shape of outputs: # (num_steps, batch_size, vocab_size) outputs = self.dense(torch.cat(outputs, dim=0)) return outputs.permute(1, 0, 2), [enc_outputs, hidden_state, enc_valid_lens] @property def attention_weights(self): return self._attention_weights
class Seq2SeqAttentionDecoder(AttentionDecoder): def __init__(self, vocab_size, embed_size, num_hiddens, num_layers, dropout=0): super().__init__() self.attention = d2l.AdditiveAttention(num_hiddens, dropout) self.embedding = nn.Embedding(vocab_size, embed_size) self.rnn = rnn.GRU(num_hiddens, num_layers, dropout=dropout) self.dense = nn.Dense(vocab_size, flatten=False) self.initialize(init.Xavier()) def init_state(self, enc_outputs, enc_valid_lens): # Shape of outputs: (num_steps, batch_size, num_hiddens). # Shape of hidden_state: (num_layers, batch_size, num_hiddens) outputs, hidden_state = enc_outputs return (outputs.swapaxes(0, 1), hidden_state, enc_valid_lens) def forward(self, X, state): # Shape of enc_outputs: (batch_size, num_steps, num_hiddens). # Shape of hidden_state: (num_layers, batch_size, num_hiddens) enc_outputs, hidden_state, enc_valid_lens = state # Shape of the output X: (num_steps, batch_size, embed_size) X = self.embedding(X).swapaxes(0, 1) outputs, self._attention_weights = [], [] for x in X: # Shape of query: (batch_size, 1, num_hiddens) query = np.expand_dims(hidden_state[-1], axis=1) # Shape of context: (batch_size, 1, num_hiddens) context = self.attention( query, enc_outputs, enc_outputs, enc_valid_lens) # Concatenate on the feature dimension x = np.concatenate((context, np.expand_dims(x, axis=1)), axis=-1) # Reshape x as (1, batch_size, embed_size + num_hiddens) out, hidden_state = self.rnn(x.swapaxes(0, 1), hidden_state) hidden_state = hidden_state[0] outputs.append(out) self._attention_weights.append(self.attention.attention_weights) # After fully connected layer transformation, shape of outputs: # (num_steps, batch_size, vocab_size) outputs = self.dense(np.concatenate(outputs, axis=0)) return outputs.swapaxes(0, 1), [enc_outputs, hidden_state, enc_valid_lens] @property def attention_weights(self): return self._attention_weights
class Seq2SeqAttentionDecoder(nn.Module): vocab_size: int embed_size: int num_hiddens: int num_layers: int dropout: float = 0 def setup(self): self.attention = d2l.AdditiveAttention(self.num_hiddens, self.dropout) self.embedding = nn.Embed(self.vocab_size, self.embed_size) self.dense = nn.Dense(self.vocab_size) self.rnn = d2l.GRU(num_hiddens, num_layers, dropout=self.dropout) def init_state(self, enc_outputs, enc_valid_lens, *args): # Shape of outputs: (num_steps, batch_size, num_hiddens). # Shape of hidden_state: (num_layers, batch_size, num_hiddens) outputs, hidden_state = enc_outputs # Attention Weights are returned as part of state; init with None return (outputs.transpose(1, 0, 2), hidden_state, enc_valid_lens) @nn.compact def __call__(self, X, state, training=False): # Shape of enc_outputs: (batch_size, num_steps, num_hiddens). # Shape of hidden_state: (num_layers, batch_size, num_hiddens) # Ignore Attention value in state enc_outputs, hidden_state, enc_valid_lens = state # Shape of the output X: (num_steps, batch_size, embed_size) X = self.embedding(X).transpose(1, 0, 2) outputs, attention_weights = [], [] for x in X: # Shape of query: (batch_size, 1, num_hiddens) query = jnp.expand_dims(hidden_state[-1], axis=1) # Shape of context: (batch_size, 1, num_hiddens) context, attention_w = self.attention(query, enc_outputs, enc_outputs, enc_valid_lens, training=training) # Concatenate on the feature dimension x = jnp.concatenate((context, jnp.expand_dims(x, axis=1)), axis=-1) # Reshape x as (1, batch_size, embed_size + num_hiddens) out, hidden_state = self.rnn(x.transpose(1, 0, 2), hidden_state, training=training) outputs.append(out) attention_weights.append(attention_w) # Flax sow API is used to capture intermediate variables self.sow('intermediates', 'dec_attention_weights', attention_weights) # After fully connected layer transformation, shape of outputs: # (num_steps, batch_size, vocab_size) outputs = self.dense(jnp.concatenate(outputs, axis=0)) return outputs.transpose(1, 0, 2), [enc_outputs, hidden_state, enc_valid_lens]
class Seq2SeqAttentionDecoder(AttentionDecoder): def __init__(self, vocab_size, embed_size, num_hiddens, num_layers, dropout=0): super().__init__() self.attention = d2l.AdditiveAttention(num_hiddens, num_hiddens, num_hiddens, dropout) self.embedding = tf.keras.layers.Embedding(vocab_size, embed_size) self.rnn = tf.keras.layers.RNN(tf.keras.layers.StackedRNNCells( [tf.keras.layers.GRUCell(num_hiddens, dropout=dropout) for _ in range(num_layers)]), return_sequences=True, return_state=True) self.dense = tf.keras.layers.Dense(vocab_size) def init_state(self, enc_outputs, enc_valid_lens): # Shape of outputs: (batch_size, num_steps, num_hiddens). # Length of list hidden_state is num_layers, where the shape of its # element is (batch_size, num_hiddens) outputs, hidden_state = enc_outputs return (tf.transpose(outputs, (1, 0, 2)), hidden_state, enc_valid_lens) def call(self, X, state, **kwargs): # Shape of output enc_outputs: # (batch_size, num_steps, num_hiddens) # Length of list hidden_state is num_layers, where the shape of its # element is (batch_size, num_hiddens) enc_outputs, hidden_state, enc_valid_lens = state # Shape of the output X: (num_steps, batch_size, embed_size) X = self.embedding(X) # Input X has shape: (batch_size, num_steps) X = tf.transpose(X, perm=(1, 0, 2)) outputs, self._attention_weights = [], [] for x in X: # Shape of query: (batch_size, 1, num_hiddens) query = tf.expand_dims(hidden_state[-1], axis=1) # Shape of context: (batch_size, 1, num_hiddens) context = self.attention(query, enc_outputs, enc_outputs, enc_valid_lens, **kwargs) # Concatenate on the feature dimension x = tf.concat((context, tf.expand_dims(x, axis=1)), axis=-1) out = self.rnn(x, hidden_state, **kwargs) hidden_state = out[1:] outputs.append(out[0]) self._attention_weights.append(self.attention.attention_weights) # After fully connected layer transformation, shape of outputs: # (batch_size, num_steps, vocab_size) outputs = self.dense(tf.concat(outputs, axis=1)) return outputs, [enc_outputs, hidden_state, enc_valid_lens] @property def attention_weights(self): return self._attention_weights
在下文中,我们使用 4 个序列的小批量测试实施的解码器,每个序列有 7 个时间步长。
vocab_size, embed_size, num_hiddens, num_layers = 10, 8, 16, 2 batch_size, num_steps = 4, 7 encoder = d2l.Seq2SeqEncoder(vocab_size, embed_size, num_hiddens, num_layers) decoder = Seq2SeqAttentionDecoder(vocab_size, embed_size, num_hiddens, num_layers) X = torch.zeros((batch_size, num_steps), dtype=torch.long) state = decoder.init_state(encoder(X), None) output, state = decoder(X, state) d2l.check_shape(output, (batch_size, num_steps, vocab_size)) d2l.check_shape(state[0], (batch_size, num_steps, num_hiddens)) d2l.check_shape(state[1][0], (batch_size, num_hiddens))
vocab_size, embed_size, num_hiddens, num_layers = 10, 8, 16, 2 batch_size, num_steps = 4, 7 encoder = d2l.Seq2SeqEncoder(vocab_size, embed_size, num_hiddens, num_layers) decoder = Seq2SeqAttentionDecoder(vocab_size, embed_size, num_hiddens, num_layers) X = np.zeros((batch_size, num_steps)) state = decoder.init_state(encoder(X), None) output, state = decoder(X, state) d2l.check_shape(output, (batch_size, num_steps, vocab_size)) d2l.check_shape(state[0], (batch_size, num_steps, num_hiddens)) d2l.check_shape(state[1][0], (batch_size, num_hiddens))
vocab_size, embed_size, num_hiddens, num_layers = 10, 8, 16, 2 batch_size, num_steps = 4, 7 encoder = d2l.Seq2SeqEncoder(vocab_size, embed_size, num_hiddens, num_layers) decoder = Seq2SeqAttentionDecoder(vocab_size, embed_size, num_hiddens, num_layers) X = jnp.zeros((batch_size, num_steps), dtype=jnp.int32) state = decoder.init_state(encoder.init_with_output(d2l.get_key(), X, training=False)[0], None) (output, state), _ = decoder.init_with_output(d2l.get_key(), X, state, training=False) d2l.check_shape(output, (batch_size, num_steps, vocab_size)) d2l.check_shape(state[0], (batch_size, num_steps, num_hiddens)) d2l.check_shape(state[1][0], (batch_size, num_hiddens))
vocab_size, embed_size, num_hiddens, num_layers = 10, 8, 16, 2 batch_size, num_steps = 4, 7 encoder = d2l.Seq2SeqEncoder(vocab_size, embed_size, num_hiddens, num_layers) decoder = Seq2SeqAttentionDecoder(vocab_size, embed_size, num_hiddens, num_layers) X = tf.zeros((batch_size, num_steps)) state = decoder.init_state(encoder(X, training=False), None) output, state = decoder(X, state, training=False) d2l.check_shape(output, (batch_size, num_steps, vocab_size)) d2l.check_shape(state[0], (batch_size, num_steps, num_hiddens)) d2l.check_shape(state[1][0], (batch_size, num_hiddens))
11.4.3。训练
现在我们指定了新的解码器,我们可以类似于 第 10.7.6 节进行:指定超参数,实例化一个常规编码器和一个带有注意力的解码器,并训练这个模型进行机器翻译。
data = d2l.MTFraEng(batch_size=128) embed_size, num_hiddens, num_layers, dropout = 256, 256, 2, 0.2 encoder = d2l.Seq2SeqEncoder( len(data.src_vocab), embed_size, num_hiddens, num_layers, dropout) decoder = Seq2SeqAttentionDecoder( len(data.tgt_vocab), embed_size, num_hiddens, num_layers, dropout) model = d2l.Seq2Seq(encoder, decoder, tgt_pad=data.tgt_vocab[''], lr=0.005) trainer = d2l.Trainer(max_epochs=30, gradient_clip_val=1, num_gpus=1) trainer.fit(model, data)
data = d2l.MTFraEng(batch_size=128) embed_size, num_hiddens, num_layers, dropout = 256, 256, 2, 0.2 encoder = d2l.Seq2SeqEncoder( len(data.src_vocab), embed_size, num_hiddens, num_layers, dropout) decoder = Seq2SeqAttentionDecoder( len(data.tgt_vocab), embed_size, num_hiddens, num_layers, dropout) model = d2l.Seq2Seq(encoder, decoder, tgt_pad=data.tgt_vocab[''], lr=0.005) trainer = d2l.Trainer(max_epochs=30, gradient_clip_val=1, num_gpus=1) trainer.fit(model, data)
data = d2l.MTFraEng(batch_size=128) embed_size, num_hiddens, num_layers, dropout = 256, 256, 2, 0.2 encoder = d2l.Seq2SeqEncoder( len(data.src_vocab), embed_size, num_hiddens, num_layers, dropout) decoder = Seq2SeqAttentionDecoder( len(data.tgt_vocab), embed_size, num_hiddens, num_layers, dropout) model = d2l.Seq2Seq(encoder, decoder, tgt_pad=data.tgt_vocab[''], lr=0.005, training=True) trainer = d2l.Trainer(max_epochs=30, gradient_clip_val=1, num_gpus=1) trainer.fit(model, data)
data = d2l.MTFraEng(batch_size=128) embed_size, num_hiddens, num_layers, dropout = 256, 256, 2, 0.2 with d2l.try_gpu(): encoder = d2l.Seq2SeqEncoder( len(data.src_vocab), embed_size, num_hiddens, num_layers, dropout) decoder = Seq2SeqAttentionDecoder( len(data.tgt_vocab), embed_size, num_hiddens, num_layers, dropout) model = d2l.Seq2Seq(encoder, decoder, tgt_pad=data.tgt_vocab[''], lr=0.005) trainer = d2l.Trainer(max_epochs=30, gradient_clip_val=1) trainer.fit(model, data)
模型训练完成后,我们用它来将几个英语句子翻译成法语并计算它们的 BLEU 分数。
engs = ['go .', 'i lost .', 'he's calm .', 'i'm home .'] fras = ['va !', 'j'ai perdu .', 'il est calme .', 'je suis chez moi .'] preds, _ = model.predict_step( data.build(engs, fras), d2l.try_gpu(), data.num_steps) for en, fr, p in zip(engs, fras, preds): translation = [] for token in data.tgt_vocab.to_tokens(p): if token == '': break translation.append(token) print(f'{en} => {translation}, bleu,' f'{d2l.bleu(" ".join(translation), fr, k=2):.3f}')
go . => ['va', '!'], bleu,1.000 i lost . => ["j'ai", 'perdu', '.'], bleu,1.000 he's calm . => ['je', "l'ai", '.'], bleu,0.000 i'm home . => ['je', 'suis', 'chez', 'moi', '.'], bleu,1.000
engs = ['go .', 'i lost .', 'he's calm .', 'i'm home .'] fras = ['va !', 'j'ai perdu .', 'il est calme .', 'je suis chez moi .'] preds, _ = model.predict_step( data.build(engs, fras), d2l.try_gpu(), data.num_steps) for en, fr, p in zip(engs, fras, preds): translation = [] for token in data.tgt_vocab.to_tokens(p): if token == '': break translation.append(token) print(f'{en} => {translation}, bleu,' f'{d2l.bleu(" ".join(translation), fr, k=2):.3f}')
go . => ['', '!'], bleu,0.000 i lost . => ['j’ai', 'payé', '.'], bleu,0.000 he's calm . => ['je', 'suis', '', '.'], bleu,0.000 i'm home . => ['je', 'suis', 'chez', 'moi', '.'], bleu,1.000
engs = ['go .', 'i lost .', 'he's calm .', 'i'm home .'] fras = ['va !', 'j'ai perdu .', 'il est calme .', 'je suis chez moi .'] preds, _ = model.predict_step( trainer.state.params, data.build(engs, fras), data.num_steps) for en, fr, p in zip(engs, fras, preds): translation = [] for token in data.tgt_vocab.to_tokens(p): if token == '': break translation.append(token) print(f'{en} => {translation}, bleu,' f'{d2l.bleu(" ".join(translation), fr, k=2):.3f}')
go . => ['', '.'], bleu,0.000 i lost . => ["j'ai", 'perdu', '.'], bleu,1.000 he's calm . => ['je', 'suis', '', '.'], bleu,0.000 i'm home . => ['je', 'suis', 'chez', 'moi', '.'], bleu,1.000
engs = ['go .', 'i lost .', 'he's calm .', 'i'm home .'] fras = ['va !', 'j'ai perdu .', 'il est calme .', 'je suis chez moi .'] preds, _ = model.predict_step( data.build(engs, fras), d2l.try_gpu(), data.num_steps) for en, fr, p in zip(engs, fras, preds): translation = [] for token in data.tgt_vocab.to_tokens(p): if token == '': break translation.append(token) print(f'{en} => {translation}, bleu,' f'{d2l.bleu(" ".join(translation), fr, k=2):.3f}')
go . => ['', '!'], bleu,0.000 i lost . => ["j'ai", 'compris', '.'], bleu,0.000 he's calm . => ['il', 'est', 'mouillé', '.'], bleu,0.658 i'm home . => ['je', 'suis', 'parti', '.'], bleu,0.512
让我们想象一下翻译最后一个英语句子时的注意力权重。我们看到每个查询都在键值对上分配了不均匀的权重。它表明在每个解码步骤中,输入序列的不同部分被选择性地聚集在注意力池中。
_, dec_attention_weights = model.predict_step( data.build([engs[-1]], [fras[-1]]), d2l.try_gpu(), data.num_steps, True) attention_weights = torch.cat( [step[0][0][0] for step in dec_attention_weights], 0) attention_weights = attention_weights.reshape((1, 1, -1, data.num_steps)) # Plus one to include the end-of-sequence token d2l.show_heatmaps( attention_weights[:, :, :, :len(engs[-1].split()) + 1].cpu(), xlabel='Key positions', ylabel='Query positions')
_, dec_attention_weights = model.predict_step( data.build([engs[-1]], [fras[-1]]), d2l.try_gpu(), data.num_steps, True) attention_weights = np.concatenate( [step[0][0][0] for step in dec_attention_weights], 0) attention_weights = attention_weights.reshape((1, 1, -1, data.num_steps)) # Plus one to include the end-of-sequence token d2l.show_heatmaps( attention_weights[:, :, :, :len(engs[-1].split()) + 1], xlabel='Key positions', ylabel='Query positions')
_, (dec_attention_weights, _) = model.predict_step( trainer.state.params, data.build([engs[-1]], [fras[-1]]), data.num_steps, True) attention_weights = jnp.concatenate( [step[0][0][0] for step in dec_attention_weights], 0) attention_weights = attention_weights.reshape((1, 1, -1, data.num_steps)) # Plus one to include the end-of-sequence token d2l.show_heatmaps(attention_weights[:, :, :, :len(engs[-1].split()) + 1], xlabel='Key positions', ylabel='Query positions')
_, dec_attention_weights = model.predict_step( data.build([engs[-1]], [fras[-1]]), d2l.try_gpu(), data.num_steps, True) attention_weights = tf.concat( [step[0][0][0] for step in dec_attention_weights], 0) attention_weights = tf.reshape(attention_weights, (1, 1, -1, data.num_steps)) # Plus one to include the end-of-sequence token d2l.show_heatmaps(attention_weights[:, :, :, :len(engs[-1].split()) + 1], xlabel='Key positions', ylabel='Query positions')
11.4.4。概括
在预测标记时,如果并非所有输入标记都相关,则具有 Bahdanau 注意力机制的 RNN 编码器-解码器会选择性地聚合输入序列的不同部分。这是通过将状态(上下文变量)视为附加注意力池的输出来实现的。在 RNN encoder-decoder 中,Bahdanau attention 机制将前一个时间步的解码器隐藏状态视为查询,将所有时间步的编码器隐藏状态视为键和值。
11.4.5。练习
实验中用 LSTM 替换 GRU。
修改实验以用缩放的点积替换附加注意力评分函数。对训练效率有何影响?
-
pytorch
+关注
关注
2文章
808浏览量
13249
发布评论请先 登录
相关推荐
注意力机制的诞生、方法及几种常见模型
注意力机制或将是未来机器学习的核心要素
基于注意力机制的深度学习模型AT-DPCNN
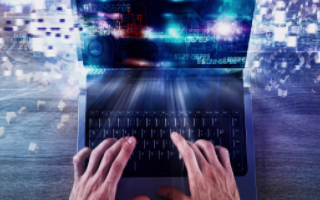
评论