在第 7 节中,我们研究了使用二维 CNN 处理二维图像数据的机制,这些机制应用于相邻像素等局部特征。尽管最初是为计算机视觉设计的,但 CNN 也广泛用于自然语言处理。简单地说,只需将任何文本序列视为一维图像即可。通过这种方式,一维 CNN 可以处理局部特征,例如n- 文本中的克。
在本节中,我们将使用textCNN模型来演示如何设计用于表示单个文本的 CNN 架构 ( Kim, 2014 )。与图 16.2.1使用带有 GloVe 预训练的 RNN 架构进行情感分析相比,图 16.3.1的唯一区别在于架构的选择。
图 16.3.1本节将预训练的 GloVe 提供给基于 CNN 的架构以进行情感分析。
import torch from torch import nn from d2l import torch as d2l batch_size = 64 train_iter, test_iter, vocab = d2l.load_data_imdb(batch_size)
from mxnet import gluon, init, np, npx from mxnet.gluon import nn from d2l import mxnet as d2l npx.set_np() batch_size = 64 train_iter, test_iter, vocab = d2l.load_data_imdb(batch_size)
16.3.1。一维卷积
在介绍模型之前,让我们看看一维卷积是如何工作的。请记住,这只是基于互相关运算的二维卷积的特例。
图 16.3.2一维互相关运算。阴影部分是第一个输出元素以及用于输出计算的输入和核张量元素: 0×1+1×2=2.
如图 16.3.2所示,在一维情况下,卷积窗口在输入张量上从左向右滑动。在滑动过程中,输入子张量(例如,0和1在 图 16.3.2中)包含在某个位置的卷积窗口和内核张量(例如,1和2在 图 16.3.2中)按元素相乘。这些乘法的总和给出单个标量值(例如, 0×1+1×2=2在图 16.3.2中)在输出张量的相应位置。
我们在以下函数中实现一维互相关 corr1d。给定一个输入张量X和一个内核张量 K,它返回输出张量Y。
def corr1d(X, K): w = K.shape[0] Y = torch.zeros((X.shape[0] - w + 1)) for i in range(Y.shape[0]): Y[i] = (X[i: i + w] * K).sum() return Y
def corr1d(X, K): w = K.shape[0] Y = np.zeros((X.shape[0] - w + 1)) for i in range(Y.shape[0]): Y[i] = (X[i: i + w] * K).sum() return Y
我们可以从 图 16.3.2构造输入张量X和核张量来验证上述一维互相关实现的输出。K
X, K = torch.tensor([0, 1, 2, 3, 4, 5, 6]), torch.tensor([1, 2]) corr1d(X, K)
tensor([ 2., 5., 8., 11., 14., 17.])
X, K = np.array([0, 1, 2, 3, 4, 5, 6]), np.array([1, 2]) corr1d(X, K)
array([ 2., 5., 8., 11., 14., 17.])
对于任何具有多个通道的一维输入,卷积核需要具有相同数量的输入通道。然后对于每个通道,对输入的一维张量和卷积核的一维张量进行互相关运算,将所有通道的结果相加得到一维输出张量。图 16.3.3显示了具有 3 个输入通道的一维互相关运算。
图 16.3.3具有 3 个输入通道的一维互相关操作。阴影部分是第一个输出元素以及用于输出计算的输入和核张量元素: 0×1+1×2+1×3+2×4+2×(−1)+3×(−3)=2.
我们可以对多个输入通道进行一维互相关运算,并验证 图 16.3.3中的结果。
def corr1d_multi_in(X, K): # First, iterate through the 0th dimension (channel dimension) of `X` and # `K`. Then, add them together return sum(corr1d(x, k) for x, k in zip(X, K)) X = torch.tensor([[0, 1, 2, 3, 4, 5, 6], [1, 2, 3, 4, 5, 6, 7], [2, 3, 4, 5, 6, 7, 8]]) K = torch.tensor([[1, 2], [3, 4], [-1, -3]]) corr1d_multi_in(X, K)
tensor([ 2., 8., 14., 20., 26., 32.])
def corr1d_multi_in(X, K): # First, iterate through the 0th dimension (channel dimension) of `X` and # `K`. Then, add them together return sum(corr1d(x, k) for x, k in zip(X, K)) X = np.array([[0, 1, 2, 3, 4, 5, 6], [1, 2, 3, 4, 5, 6, 7], [2, 3, 4, 5, 6, 7, 8]]) K = np.array([[1, 2], [3, 4], [-1, -3]]) corr1d_multi_in(X, K)
array([ 2., 8., 14., 20., 26., 32.])
请注意,多输入通道一维互相关等同于单输入通道二维互相关。为了说明,图 16.3.3中的多输入通道一维互相关的等效形式是图 16.3.4中的单输入通道二维互相关 ,其中卷积核必须与输入张量相同。
图 16.3.4单输入通道的二维互相关运算。阴影部分是第一个输出元素以及用于输出计算的输入和核张量元素: 2×(−1)+3×(−3)+1×3+2×4+0×1+1×2=2.
图 16.3.2和 图 16.3.3中的输出都只有一个通道。与第 7.4.2 节中描述的具有多个输出通道的二维卷积相同 ,我们也可以为一维卷积指定多个输出通道。
16.3.2。最大超时池化
同样,我们可以使用池化从序列表示中提取最高值作为跨时间步长的最重要特征。textCNN 中使用的最大 随时间池化与一维全局最大池化类似(Collobert等人,2011 年)。对于每个通道在不同时间步存储值的多通道输入,每个通道的输出是该通道的最大值。请注意,max-over-time 池允许在不同的通道上使用不同数量的时间步长。
16.3.3。textCNN 模型
使用一维卷积和最大时间池化,textCNN 模型将单独的预训练标记表示作为输入,然后为下游应用获取和转换序列表示。
对于单个文本序列n代表的代币 d维向量,输入张量的宽度、高度和通道数是n,1, 和d, 分别。textCNN 模型将输入转换为输出,如下所示:
定义多个一维卷积核,分别对输入进行卷积运算。具有不同宽度的卷积核可以捕获不同数量的相邻标记之间的局部特征。
对所有输出通道执行 max-over-time 池化,然后将所有标量池化输出连接为一个向量。
使用全连接层将串联向量转换为输出类别。Dropout 可用于减少过度拟合。
图 16.3.5 textCNN 的模型架构。
图 16.3.5用一个具体的例子说明了 textCNN 的模型架构。输入是一个包含 11 个标记的句子,其中每个标记由一个 6 维向量表示。所以我们有一个宽度为 11 的 6 通道输入。定义两个宽度为 2 和 4 的一维卷积核,分别具有 4 和 5 个输出通道。它们产生 4 个宽度为11−2+1=10和 5 个宽度输出通道11−4+1=8. 尽管这 9 个通道的宽度不同,但 max-over-time 池化给出了一个串联的 9 维向量,最终将其转换为用于二进制情感预测的 2 维输出向量。
16.3.3.1。定义模型
我们在下面的类中实现了 textCNN 模型。与16.2节中的双向RNN模型相比,除了用卷积层代替循环层外,我们还使用了两个嵌入层:一个具有可训练的权重,另一个具有固定的权重。
class TextCNN(nn.Module): def __init__(self, vocab_size, embed_size, kernel_sizes, num_channels, **kwargs): super(TextCNN, self).__init__(**kwargs) self.embedding = nn.Embedding(vocab_size, embed_size) # The embedding layer not to be trained self.constant_embedding = nn.Embedding(vocab_size, embed_size) self.dropout = nn.Dropout(0.5) self.decoder = nn.Linear(sum(num_channels), 2) # The max-over-time pooling layer has no parameters, so this instance # can be shared self.pool = nn.AdaptiveAvgPool1d(1) self.relu = nn.ReLU() # Create multiple one-dimensional convolutional layers self.convs = nn.ModuleList() for c, k in zip(num_channels, kernel_sizes): self.convs.append(nn.Conv1d(2 * embed_size, c, k)) def forward(self, inputs): # Concatenate two embedding layer outputs with shape (batch size, no. # of tokens, token vector dimension) along vectors embeddings = torch.cat(( self.embedding(inputs), self.constant_embedding(inputs)), dim=2) # Per the input format of one-dimensional convolutional layers, # rearrange the tensor so that the second dimension stores channels embeddings = embeddings.permute(0, 2, 1) # For each one-dimensional convolutional layer, after max-over-time # pooling, a tensor of shape (batch size, no. of channels, 1) is # obtained. Remove the last dimension and concatenate along channels encoding = torch.cat([ torch.squeeze(self.relu(self.pool(conv(embeddings))), dim=-1) for conv in self.convs], dim=1) outputs = self.decoder(self.dropout(encoding)) return outputs
class TextCNN(nn.Block): def __init__(self, vocab_size, embed_size, kernel_sizes, num_channels, **kwargs): super(TextCNN, self).__init__(**kwargs) self.embedding = nn.Embedding(vocab_size, embed_size) # The embedding layer not to be trained self.constant_embedding = nn.Embedding(vocab_size, embed_size) self.dropout = nn.Dropout(0.5) self.decoder = nn.Dense(2) # The max-over-time pooling layer has no parameters, so this instance # can be shared self.pool = nn.GlobalMaxPool1D() # Create multiple one-dimensional convolutional layers self.convs = nn.Sequential() for c, k in zip(num_channels, kernel_sizes): self.convs.add(nn.Conv1D(c, k, activation='relu')) def forward(self, inputs): # Concatenate two embedding layer outputs with shape (batch size, no. # of tokens, token vector dimension) along vectors embeddings = np.concatenate(( self.embedding(inputs), self.constant_embedding(inputs)), axis=2) # Per the input format of one-dimensional convolutional layers, # rearrange the tensor so that the second dimension stores channels embeddings = embeddings.transpose(0, 2, 1) # For each one-dimensional convolutional layer, after max-over-time # pooling, a tensor of shape (batch size, no. of channels, 1) is # obtained. Remove the last dimension and concatenate along channels encoding = np.concatenate([ np.squeeze(self.pool(conv(embeddings)), axis=-1) for conv in self.convs], axis=1) outputs = self.decoder(self.dropout(encoding)) return outputs
让我们创建一个 textCNN 实例。它有 3 个卷积层,内核宽度分别为 3、4 和 5,都有 100 个输出通道。
embed_size, kernel_sizes, nums_channels = 100, [3, 4, 5], [100, 100, 100] devices = d2l.try_all_gpus() net = TextCNN(len(vocab), embed_size, kernel_sizes, nums_channels) def init_weights(module): if type(module) in (nn.Linear, nn.Conv1d): nn.init.xavier_uniform_(module.weight) net.apply(init_weights);
embed_size, kernel_sizes, nums_channels = 100, [3, 4, 5], [100, 100, 100] devices = d2l.try_all_gpus() net = TextCNN(len(vocab), embed_size, kernel_sizes, nums_channels) net.initialize(init.Xavier(), ctx=devices)
16.3.3.2。加载预训练词向量
与第 16.2 节相同,我们加载预训练的 100 维 GloVe 嵌入作为初始化的标记表示。这些令牌表示(嵌入权重)将在 中进行训练embedding和固定constant_embedding。
glove_embedding = d2l.TokenEmbedding('glove.6b.100d') embeds = glove_embedding[vocab.idx_to_token] net.embedding.weight.data.copy_(embeds) net.constant_embedding.weight.data.copy_(embeds) net.constant_embedding.weight.requires_grad = False
glove_embedding = d2l.TokenEmbedding('glove.6b.100d') embeds = glove_embedding[vocab.idx_to_token] net.embedding.weight.set_data(embeds) net.constant_embedding.weight.set_data(embeds) net.constant_embedding.collect_params().setattr('grad_req', 'null')
16.3.3.3。训练和评估模型
现在我们可以训练用于情感分析的 textCNN 模型。
lr, num_epochs = 0.001, 5 trainer = torch.optim.Adam(net.parameters(), lr=lr) loss = nn.CrossEntropyLoss(reduction="none") d2l.train_ch13(net, train_iter, test_iter, loss, trainer, num_epochs, devices)
loss 0.067, train acc 0.978, test acc 0.869 2827.9 examples/sec on [device(type='cuda', index=0), device(type='cuda', index=1)]
lr, num_epochs = 0.001, 5 trainer = gluon.Trainer(net.collect_params(), 'adam', {'learning_rate': lr}) loss = gluon.loss.SoftmaxCrossEntropyLoss() d2l.train_ch13(net, train_iter, test_iter, loss, trainer, num_epochs, devices)
loss 0.089, train acc 0.970, test acc 0.867 1527.8 examples/sec on [gpu(0), gpu(1)]
下面我们使用经过训练的模型来预测两个简单句子的情绪。
d2l.predict_sentiment(net, vocab, 'this movie is so great')
'positive'
d2l.predict_sentiment(net, vocab, 'this movie is so bad')
'negative'
d2l.predict_sentiment(net, vocab, 'this movie is so great')
'positive'
d2l.predict_sentiment(net, vocab, 'this movie is so bad')
'negative'
16.3.4。概括
一维 CNN 可以处理局部特征,例如 n- 文本中的克。
多输入通道一维互相关等价于单输入通道二维互相关。
max-over-time 池允许在不同的通道上使用不同数量的时间步长。
textCNN 模型使用一维卷积层和 max-over-time 池化层将单个标记表示转换为下游应用程序输出。
16.3.5。练习
调整超参数并比较16.2 节和本节中用于情感分析的两种架构,例如分类精度和计算效率。
你能否利用16.2节习题中介绍的方法进一步提高模型的分类准确率 ?
在输入表示中添加位置编码。它会提高分类精度吗?
-
神经网络
+关注
关注
42文章
4771浏览量
100754 -
卷积
+关注
关注
0文章
95浏览量
18509 -
pytorch
+关注
关注
2文章
808浏览量
13221
发布评论请先 登录
相关推荐
使用PyTorch深度解析卷积神经网络
卷积神经网络如何使用
卷积神经网络模型发展及应用
基于深度学习的卷积神经网络模型分析文本的情感倾向
PyTorch教程8.1之深度卷积神经网络(AlexNet)
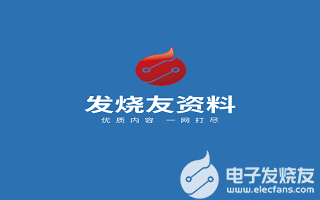
评论