来源:古月居
前言
KNN(k-Nearest Neighbors)思想简单,应用的数学知识几乎为0,所以作为机器学习的入门非常实用、可以解释机器学习算法使用过程中的很多细节问题。能够更加完整地刻画机器学习应用的流程。
首先大致介绍一下KNN的思想,假设我们现在有两类数据集,一类是红色的点表示,另一类用蓝色的点表示,这两类点就作为我们的训练数据集,当有一个新的数据绿色的点,那么我们该怎么给这个绿色的点进行分类呢?
一般情况下,我们需要先指定一个k,当一个新的数据集来临时,我们首先计算这个新的数据跟训练集中的每一个数据的距离,一般使用欧氏距离。
然后从中选出距离最近的k个点,这个k一般选取为奇数,方便后面投票决策。在k个点中根据最多的确定新的数据属于哪一类。
KNN基础
1.先创建好数据集x_train, y_train,和一个新的数据x_new, 并使用matplot将其可视化出来。
import numpy as np import matplotlib.pyplot as plt raw_data_x = [[3.3935, 2.3313], [3.1101, 1.7815], [1.3438, 3.3684], [3.5823, 4.6792], [2.2804, 2.8670], [7.4234, 4.6965], [5.7451, 3.5340], [9.1722, 2.5111], [7.7928, 3.4241], [7.9398, 0.7916]] raw_data_y = [0, 0, 0, 0, 0, 1, 1, 1, 1, 1] x_train = np.array(raw_data_x) y_train = np.array(raw_data_y) x_new = np.array([8.0936, 3.3657]) plt.scatter(x_train[y_train==0,0], x_train[y_train==0,1], color='g') plt.scatter(x_train[y_train==1,0], x_train[y_train==1,1], color='r') plt.scatter(x_new[0], x_new[1], color='b') plt.show()
1.knn过程
2.计算距离
from math import sqrt distance = [] for x in x_train: d = sqrt(np.sum((x_new - x) ** 2)) distance.append(d) # 其实上面这些代码用一行就可以搞定 # distances = [sqrt(np.sum((x_new - x) ** 2)) for x in x_train]
输出结果:
[10.888422144185997, 11.825242797930196, 15.18734646375067, 11.660703691887552, 12.89974598548359, 12.707715895864213, 9.398411207752083, 15.62480440229573, 12.345673749536719, 14.394770082568183]
将距离进行排序,返回的是排序之后的索引位置
nearsest = np.argsort(distances)
输出结果:array([6, 0, 3, 1, 8, 5, 4, 9, 2, 7], dtype=int64)
取k个点,假设k=5
k = 5 topk_y = [y_train[i] for i in nearest[:k]] topk_y
输出结果:[1, 0, 0, 0, 1]
根据输出结果我们可以发现,新来的数据距离最近的5个点,有三个点属于第一类,有两个点属于第二类,根据少数服从多数原则,新来的数据就属于第一类!
投票
from collections import Counter Counter(topk_y)
输出结果:Counter({1: 2, 0: 3})
votes = Counter(topk_y) votes.most_common(1) y_new = votes.most_common(1)[0][0]
输出结果:0
这样,我们就完成了一个基本的knn!
自己写一个knn函数
knn是一个不需要训练过程的机器学习算法。其数据集可以近似看成一个模型。
import numpy as np from math import sqrt from collections import Counter def kNN_classifier(k, x_train, y_train, x_new): assert 1 <= k <= x_train.shape[0], "k must be valid" assert x_train.shape[0] == y_train.shape[0], "the size of x_train must be equal to the size of y_train" assert x_train.shape[1] == x_new.shape[0], "the feature number of x_new must be equal to x_train" distances = [sqrt(np.sum((x_new - x) ** 2)) for x in x_train] nearest = np.argsort(distances) topk_y = [y_train[i] for i in nearest[:k]] votes = Counter(topk_y) return votes.most_common(1)[0][0]
测试一下:
raw_data_x = [[3.3935, 2.3313], [3.1101, 1.7815], [1.3438, 3.3684], [3.5823, 4.6792], [2.2804, 2.8670], [7.4234, 4.6965], [5.7451, 3.5340], [9.1722, 2.5111], [7.7928, 3.4241], [7.9398, 0.7916]] raw_data_y = [0, 0, 0, 0, 0, 1, 1, 1, 1, 1] x_train = np.array(raw_data_x) y_train = np.array(raw_data_y) x_new = np.array([8.0936, 3.3657]) y_new = kNN_classifier(5, x_train, y_train, x_new) print(y_new)
使用sklearn中的KNN
from sklearn.neighbors import KNeighborsClassifier import numpy as np raw_data_x = [[3.3935, 2.3313], [3.1101, 1.7815], [1.3438, 3.3684], [3.5823, 4.6792], [2.2804, 2.8670], [7.4234, 4.6965], [5.7451, 3.5340], [9.1722, 2.5111], [7.7928, 3.4241], [7.9398, 0.7916]] raw_data_y = [0, 0, 0, 0, 0, 1, 1, 1, 1, 1] x_train = np.array(raw_data_x) y_train = np.array(raw_data_y) x_new = np.array([8.0936, 3.3657]) knn_classifier = KNeighborsClassifier(n_neighbors=5) knn_classifier.fit(x_train, y_train) y_new = knn_classifier.predict(x_new.reshape(1, -1)) print(y_new[0])
自己写一个面向对象的KNN
import numpy as np from math import sqrt from collections import Counter class KNNClassifier(): def __init__(self, k): assert 1 <= k, "k must be valid" self.k = k self._x_train = None self._y_train = None def fit(self, x_train, y_train): assert x_train.shape[0] == y_train.shape[0], "the size of x_train must be equal to the size of y_train" assert self.k <= x_train.shape[0], "the size of x_train must be at least k" self._x_train = x_train self._y_train = y_train return self def predict(self, x_new): x_new = x_new.reshape(1, -1) assert self._x_train is not None and self._y_train is not None, "must fit before predict" assert x_new.shape[1] == self._x_train.shape[1], "the feature number of x must be equal to x_train" y_new = [self._predict(x) for x in x_new] return np.array(y_new) def _predict(self, x): assert x.shape[0] == self._x_train.shape[1], "the feature number of x must be equal to x_train" distances = [sqrt(np.sum((x_train - x) ** 2)) for x_train in self._x_train] nearest = np.argsort(distances) topk_y = [self._y_train[i] for i in nearest[:self.k]] votes = Counter(topk_y) return votes.most_common(1)[0][0] def __repr__(self): return "KNN(k=%d)" % self.k
测试一下:
raw_data_x = [[3.3935, 2.3313], [3.1101, 1.7815], [1.3438, 3.3684], [3.5823, 4.6792], [2.2804, 2.8670], [7.4234, 4.6965], [5.7451, 3.5340], [9.1722, 2.5111], [7.7928, 3.4241], [7.9398, 0.7916]] raw_data_y = [0, 0, 0, 0, 0, 1, 1, 1, 1, 1] x_train = np.array(raw_data_x) y_train = np.array(raw_data_y) x_new = np.array([8.0936, 3.3657]) knn_clf = KNNClassifier(6) knn_clf.fit(x_train, y_train) y_new = knn_clf.predict(x_new) print(y_new[0])
分割数据集
import numpy as np from sklearn import datasets def train_test_split(x, y, test_ratio=0.2, seed=None): assert x.shape[0] == y.shape[0], "the size of x must be equal to the size of y" assert 0.0 <= test_ratio <= 1.0, "test_ratio must be valid" if seed: np.random.seed(seed) shuffle_idx = np.random.permutation(len(x)) test_size = int(len(x) * test_ratio) test_idx = shuffle_idx[:test_size] train_idx = shuffle_idx[test_size:] x_train = x[train_idx] y_train = y[train_idx] x_test = x[test_idx] y_test = y[test_idx] return x_train, y_train, x_test, y_test
sklearn中鸢尾花数据测试KNN
import numpy as np from sklearn import datasets from knn_clf import KNNClassifier iris = datasets.load_iris() x = iris.data y = iris.target x_train, y_train, x_test, y_test = train_test_split(x, y) my_knn_clf = KNNClassifier(k=3) my_knn_clf.fit(x_train, y_train) y_predict = my_knn_clf.predict(x_test) print(sum(y_predict == y_test)) print(sum(y_predict == y_test) / len(y_test)) # 也可以使用sklearn中自带的数据集拆分方法 from sklearn.model_selection import train_test_split import numpy as np from sklearn import datasets from knn_clf import KNNClassifier iris = datasets.load_iris() x = iris.data y = iris.target x_train, y_train, x_test, y_test = train_test_split(x, y, test_size=0.2, random_state=666) my_knn_clf = KNNClassifier(k=3) my_knn_clf.fit(x_train, y_train) y_predict = my_knn_clf.predict(x_test) print(sum(y_predict == y_test)) print(sum(y_predict == y_test) / len(y_test))
sklearn中手写数字数据集测试KNN
首先,先来了解一下手写数字数据集。
import numpy as np import matplotlib.pyplot as plt from sklearn import datasets digits = datasets.load_digits() digits.keys() print(digits.DESCR) y.shape digits.target_names y[:100] x[:10] some_digit = x[666] y[666] some_digit_image = some_digit.reshape(8, 8) plt.imshow(some_digit_image, cmap=plt.cm.binary) plt.show()
接下来,就开始动手试试。
from sklearn import datasets from shuffle_dataset import train_test_split from knn_clf import KNNClassifier digits = datasets.load_digits() x = digits.data y = digits.target x_train, y_train, x_test, y_test = train_test_split(x, y, test_ratio=0.2) my_knn_clf = KNNClassifier(k=3) my_knn_clf.fit(x_train, y_train) y_predict = my_knn_clf.predict(x_test) print(sum(y_predict == y_test) / len(y_test))
把求acc封装成一个函数,方便调用。
def accuracy_score(y_true, y_predict): assert y_true.shape[0] == y_predict.shape[0], "the size of y_true must be equal to the size of y_predict" return sum(y_true == y_predict) / len(y_true)
接下来把它封装到KNNClassifier的类中。
import numpy as np from math import sqrt from collections import Counter from metrics import accuracy_score class KNNClassifier(): def __init__(self, k): assert 1 <= k, "k must be valid" self.k = k self._x_train = None self._y_train = None def fit(self, x_train, y_train): assert x_train.shape[0] == y_train.shape[0], "the size of x_train must be equal to the size of y_train" assert self.k <= x_train.shape[0], "the size of x_train must be at least k" self._x_train = x_train self._y_train = y_train return self def predict(self, x_new): # x_new = x_new.reshape(1, -1) assert self._x_train is not None and self._y_train is not None, "must fit before predict" assert x_new.shape[1] == self._x_train.shape[1], "the feature number of x must be equal to x_train" y_new = [self._predict(x) for x in x_new] return np.array(y_new) def _predict(self, x): assert x.shape[0] == self._x_train.shape[1], "the feature number of x must be equal to x_train" distances = [sqrt(np.sum((x_train - x) ** 2)) for x_train in self._x_train] nearest = np.argsort(distances) topk_y = [self._y_train[i] for i in nearest[:self.k]] votes = Counter(topk_y) return votes.most_common(1)[0][0] def score(self, x_test, y_test): y_predict = self.predict(x_test) return accuracy_score(y_test, y_predict) def __repr__(self): return "KNN(k=%d)" % self.k
其实,在sklearn中这些都已经封装好了。
from sklearn.metrics import accuracy_score from sklearn.model_selection import train_test_split from sklearn.neighbors import KNeighborsClassifier digits = datasets.load_digits() x = digits.data y = digits.target x_train, x_test, y_train, y_test = train_test_split(x, y, test_size=0.2) knn_classifier = KNeighborsClassifier(n_neighbors=3) knn_classifier.fit(x_train, y_train) knn_classifier.score(x_test, y_test)
超参数
k
在knn中的超参数k何时最优?
from sklearn.metrics import accuracy_score from sklearn.model_selection import train_test_split from sklearn.neighbors import KNeighborsClassifier digits = datasets.load_digits() x = digits.data y = digits.target x_train, x_test, y_train, y_test = train_test_split(x, y, test_size=0.2) best_score = 0.0 best_k = -1 for k in range(1, 11): knn_clf = KNeighborsClassifier(n_neighbors=k) knn_clf.fit(x_train, y_train) score = knn_clf.score(x_test, y_test) if score > best_score: best_k = k best_score = score print("best k=", best_k) print("best score=", best_score)
投票方式
上面这张图,绿色的球最近的三颗球分别是红色的1号,紫色的3号和蓝色的4号。如果只考虑绿色的k个近邻中多数服从少数,目前来说就是平票。
即使不是平票,红色也是距离绿色最近。此时我们就可以考虑给他们加个权重。一般使用距离的倒数作为权重。假设距离分别为1、 3、 4
红球:1 紫+蓝:1/3 + 1/4 = 7/12
这两者加起来都没有红色的权重大,因此最终将这颗绿球归为红色类别。这样能有效解决平票问题。 因此,这也算knn的一个超参数。
其实这个在sklearn封装的knn中已经考虑到了这个问题。在KNeighborsClassifier(n_neighbors=k,weights=?)
还有一个参数weights,一般有两种:uniform、distance。
from sklearn.metrics import accuracy_score from sklearn.model_selection import train_test_split from sklearn.neighbors import KNeighborsClassifier digits = datasets.load_digits() x = digits.data y = digits.target x_train, x_test, y_train, y_test = train_test_split(x, y, test_size=0.2) best_method = "" best_score = 0.0 best_k = -1 for method in["uniform", "distance"]: for k in range(1, 11): knn_clf = KNeighborsClassifier(n_neighbors=k, weights=method) knn_clf.fit(x_train, y_train) score = knn_clf.score(x_test, y_test) if score > best_score: best_method = method best_k = k best_score = score print("best_method=", best_method) print("best k=", best_k) print("best score=", best_score)
p
如果使用距离,那么有很多种距离可以使用,欧氏距离、曼哈顿距离、明可夫斯基距离。
from sklearn.metrics import accuracy_score from sklearn.model_selection import train_test_split from sklearn.neighbors import KNeighborsClassifier digits = datasets.load_digits() x = digits.data y = digits.target x_train, x_test, y_train, y_test = train_test_split(x, y, test_size=0.2) best_p = -1 best_score = 0.0 best_k = -1 for p in range(1, 6): for k in range(1, 11): knn_clf = KNeighborsClassifier(n_neighbors=k, weights="distance", p=p) knn_clf.fit(x_train, y_train) score = knn_clf.score(x_test, y_test) if score > best_score: best_p = p best_k = k best_score = score print("best_p=", best_p) print("best k=", best_k) print("best score=", best_score)
-
算法
+关注
关注
23文章
4599浏览量
92617 -
代码
+关注
关注
30文章
4741浏览量
68324 -
机器学习
+关注
关注
66文章
8373浏览量
132391 -
数据集
+关注
关注
4文章
1205浏览量
24635
发布评论请先 登录
相关推荐
【下载】《机器学习》+《机器学习实战》
基于建构主义学习理论的藏文音素拼读法MCAI设计
机器学习理论基础介绍
基于k近邻的完全随机森林算法KCRForest
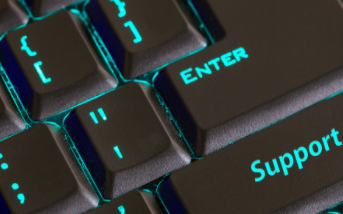
评论