前文
TensorRT-LLM正式出来有半个月了,一直没有时间玩,周末趁着有时间跑一下。
之前玩内测版的时候就需要cuda-12.x,正式出来仍是需要cuda-12.x,主要是因为tensorr-llm中依赖的CUBIN(二进制代码)是基于cuda12.x编译生成的,想要跑只能更新驱动。
I’ve verified with our CUDA team. A CUBIN built with CUDA 12.x will not load in CUDA 11.x. CUDA 12.x is required to use TensorRT-LLM.
因此,想要快速跑TensorRT-LLM,建议直接将nvidia-driver升级到535.xxx,利用docker跑即可,省去自己折腾环境,至于想要自定义修改源码,也在docker中搞就可以。
理论上替换原始代码中的该部分就可以使用别的cuda版本了(batch manager只是不开源,和cuda版本应该没关系,主要是FMA模块,另外TensorRT-llm依赖的TensorRT有cuda11.x版本,配合inflight_batcher_llm跑的triton-inference-server也和cuda12.x没有强制依赖关系):
tensorrt-llm中预先编译好的部分
说完环境要求,开始配环境吧!
搭建运行环境以及库
首先拉取镜像,宿主机显卡驱动需要高于等于535:
docker pull nvcr.io/nvidia/tritonserver:23.10-trtllm-python-py3
这个镜像是前几天刚出的,包含了运行TensorRT-LLM的所有环境(TensorRT、mpi、nvcc、nccl库等等),省去自己配环境的烦恼。
拉下来镜像后,启动镜像:
dockerrun-it-d--cap-add=SYS_PTRACE--cap-add=SYS_ADMIN--security-optseccomp=unconfined--gpus=all--shm-size=16g--privileged--ulimitmemlock=-1--name=developnvcr.io/nvidia/tritonserver:23.10-trtllm-python-py3bash
接下来的操作全在这个容器里。
编译tensorrt-llm
首先获取git仓库,因为这个镜像中只有运行需要的lib,模型还是需要自行编译的(因为依赖的TensorRT,用过trt的都知道需要构建engine),所以首先编译tensorrRT-LLM:
#TensorRT-LLMusesgit-lfs,whichneedstobeinstalledinadvance. apt-getupdate&&apt-get-yinstallgitgit-lfs gitclonehttps://github.com/NVIDIA/TensorRT-LLM.git cdTensorRT-LLM gitsubmoduleupdate--init--recursive gitlfsinstall gitlfspull
然后进入仓库进行编译:
python3./scripts/build_wheel.py--trt_root/usr/local/tensorrt
一般不会有环境问题,这个docekr中已经包含了所有需要的包,执行build_wheel的时候会按照脚本中的步骤pip install一些需要的包,然后运行cmake和make编译文件:
.. adding'tensorrt_llm/tools/plugin_gen/templates/functional.py.tpl' adding'tensorrt_llm/tools/plugin_gen/templates/plugin.cpp.tpl' adding'tensorrt_llm/tools/plugin_gen/templates/plugin.h.tpl' adding'tensorrt_llm/tools/plugin_gen/templates/plugin_common.cpp' adding'tensorrt_llm/tools/plugin_gen/templates/plugin_common.h' adding'tensorrt_llm/tools/plugin_gen/templates/tritonPlugins.cpp.tpl' adding'tensorrt_llm-0.5.0.dist-info/LICENSE' adding'tensorrt_llm-0.5.0.dist-info/METADATA' adding'tensorrt_llm-0.5.0.dist-info/WHEEL' adding'tensorrt_llm-0.5.0.dist-info/top_level.txt' adding'tensorrt_llm-0.5.0.dist-info/zip-safe' adding'tensorrt_llm-0.5.0.dist-info/RECORD' removingbuild/bdist.linux-x86_64/wheel Successfullybuilttensorrt_llm-0.5.0-py3-none-any.whl
然后pip install tensorrt_llm-0.5.0-py3-none-any.whl即可。
运行
首先编译模型,因为最近没有下载新模型,还是拿旧的llama做例子。其实吧,其他llm也一样(chatglm、qwen等等),只要trt-llm支持,编译运行方法都一样的,在hugging face下载好要测试的模型即可。
这里我执行:
python/work/code/TensorRT-LLM/examples/llama/build.py
--model_dir/work/models/GPT/LLAMA/llama-7b-hf#可以替换为你自己的llm模型 --dtypefloat16 --remove_input_padding --use_gpt_attention_pluginfloat16 --enable_context_fmha --use_gemm_pluginfloat16 --use_inflight_batching#开启inflightbatching --output_dir/work/trtModel/llama/1-gpu
然后就是TensorRT的编译、构建engine的过程(因为使用了plugin,编译挺快的,这里我只用了一张A4000,所以没有设置world_size,默认为1),这里有很多细节,后续会聊。
编译好engine后,会生成/work/trtModel/llama/1-gpu,后续会用到。
然后克隆https://github.com/triton-inference-server/tensorrtllm_backend:
执行以下命令:
cdtensorrtllm_backend mkdirtriton_model_repo #拷贝出来模板模型文件夹 cp-rall_models/inflight_batcher_llm/*triton_model_repo/ #将刚才生成好的`/work/trtModel/llama/1-gpu`移动到模板模型文件夹中 cp/work/trtModel/llama/1-gpu/*triton_model_repo/tensorrt_llm/1
然后修改triton_model_repo/中的config:
triton_model_repo/preprocessing/config.pbtxt
Name | Description |
---|---|
tokenizer_dir | The path to the tokenizer for the model.这里我改成/work/models/GPT/LLAMA/llama-7b-hf |
tokenizer_type | The type of the tokenizer for the model, t5, auto and llama are supported. 这里我设置为'llama' |
triton_model_repo/tensorrt_llm/config.pbtxt
Name | Description |
---|---|
decoupled | Controls streaming. Decoupled mode must be set to True if using the streaming option from the client.这里我设置为 true |
gpt_model_type | Set to inflight_fused_batching when enabling in-flight batching support. To disable in-flight batching, set to V1 这里保持默认不变 |
gpt_model_path | Path to the TensorRT-LLM engines for deployment. In this example, the path should be set to /tensorrtllm_backend/triton_model_repo/tensorrt_llm/1 as the tensorrtllm_backend directory will be mounted to /tensorrtllm_backend within the container 这里改成 triton_model_repo/tensorrt_llm/1 |
triton_model_repo/postprocessing/config.pbtxt
Name | Description |
---|---|
tokenizer_dir | The path to the tokenizer for the model. In this example, the path should be set to /tensorrtllm_backend/tensorrt_llm/examples/gpt/gpt2 as the tensorrtllm_backend directory will be mounted to /tensorrtllm_backend within the container 这里改成/work/models/GPT/LLAMA/llama-7b-hf |
tokenizer_type | The type of the tokenizer for the model, t5, auto and llama are supported. In this example, the type should be set to auto 这里我是llama |
设置好之后进入tensorrtllm_backend执行:
python3scripts/launch_triton_server.py--world_size=1--model_repo=triton_model_repo
顺利的话就会输出:
root@6aaab84e59c0:/work/code/tensorrtllm_backend#I11051458.2868362561098pinned_memory_manager.cc:241]Pinnedmemorypooliscreatedat'0x7ffb76000000'withsize268435456 I11051458.2869732561098cuda_memory_manager.cc:107]CUDAmemorypooliscreatedondevice0withsize67108864 I11051458.2881202561098model_lifecycle.cc:461]loading:tensorrt_llm:1 I11051458.2881352561098model_lifecycle.cc:461]loading:preprocessing:1 I11051458.2881422561098model_lifecycle.cc:461]loading:postprocessing:1 [TensorRT-LLM][WARNING]max_tokens_in_paged_kv_cacheisnotspecified,willusedefaultvalue [TensorRT-LLM][WARNING]batch_scheduler_policyparameterwasnotfoundorisinvalid(mustbemax_utilizationorguaranteed_no_evict) [TensorRT-LLM][WARNING]kv_cache_free_gpu_mem_fractionisnotspecified,willusedefaultvalueof0.85ormax_tokens_in_paged_kv_cache [TensorRT-LLM][WARNING]max_num_sequencesisnotspecified,willbesettotheTRTenginemax_batch_size [TensorRT-LLM][WARNING]enable_trt_overlapisnotspecified,willbesettotrue [TensorRT-LLM][WARNING][json.exception.type_error.302]typemustbenumber,butisnull [TensorRT-LLM][WARNING]Optionalvalueforparametermax_num_tokenswillnotbeset. [TensorRT-LLM][INFO]InitializingMPIwiththreadmode1 I11051458.3929152561098python_be.cc:2199]TRITONBACKEND_ModelInstanceInitialize:postprocessing_0_0(CPUdevice0) I11051458.3929792561098python_be.cc:2199]TRITONBACKEND_ModelInstanceInitialize:preprocessing_0_0(CPUdevice0) [TensorRT-LLM][INFO]MPIsize:1,rank:0 I11051458.7321652561098model_lifecycle.cc:818]successfullyloaded'postprocessing' I11051459.3832552561098model_lifecycle.cc:818]successfullyloaded'preprocessing' [TensorRT-LLM][INFO]TRTGptModelmaxNumSequences:16 [TensorRT-LLM][INFO]TRTGptModelmaxBatchSize:8 [TensorRT-LLM][INFO]TRTGptModelenableTrtOverlap:1 [TensorRT-LLM][INFO]Loadedenginesize:12856MiB [TensorRT-LLM][INFO][MemUsageChange]InitcuBLAS/cuBLASLt:CPU+0,GPU+8,now:CPU13144,GPU13111(MiB) [TensorRT-LLM][INFO][MemUsageChange]InitcuDNN:CPU+2,GPU+10,now:CPU13146,GPU13121(MiB) [TensorRT-LLM][INFO][MemUsageChange]TensorRT-managedallocationinenginedeserialization:CPU+0,GPU+12852,now:CPU0,GPU12852(MiB) [TensorRT-LLM][INFO][MemUsageChange]InitcuBLAS/cuBLASLt:CPU+0,GPU+8,now:CPU13164,GPU14363(MiB) [TensorRT-LLM][INFO][MemUsageChange]InitcuDNN:CPU+0,GPU+8,now:CPU13164,GPU14371(MiB) [TensorRT-LLM][INFO][MemUsageChange]TensorRT-managedallocationinIExecutionContextcreation:CPU+0,GPU+0,now:CPU0,GPU12852(MiB) [TensorRT-LLM][INFO][MemUsageChange]InitcuBLAS/cuBLASLt:CPU+0,GPU+8,now:CPU13198,GPU14391(MiB) [TensorRT-LLM][INFO][MemUsageChange]InitcuDNN:CPU+0,GPU+10,now:CPU13198,GPU14401(MiB) [TensorRT-LLM][INFO][MemUsageChange]TensorRT-managedallocationinIExecutionContextcreation:CPU+0,GPU+0,now:CPU0,GPU12852(MiB) [TensorRT-LLM][INFO]Using2878tokensinpagedKVcache. I11051417.2992932561098model_lifecycle.cc:818]successfullyloaded'tensorrt_llm' I11051417.3036612561098model_lifecycle.cc:461]loading:ensemble:1 I11051417.3058972561098model_lifecycle.cc:818]successfullyloaded'ensemble' I11051417.3060512561098server.cc:592] +------------------+------+ |RepositoryAgent|Path| +------------------+------+ +------------------+------+ I11051417.3064012561098server.cc:619] +-------------+-----------------------------------------------------------------+------------------------------------------------------------------------------------------------------+ |Backend|Path|Config| +-------------+-----------------------------------------------------------------+------------------------------------------------------------------------------------------------------+ |tensorrtllm|/opt/tritonserver/backends/tensorrtllm/libtriton_tensorrtllm.so|{"cmdline":{"auto-complete-config":"false","backend-directory":"/opt/tritonserver/backends","min-com| |||pute-capability":"6.000000","default-max-batch-size":"4"}}| |python|/opt/tritonserver/backends/python/libtriton_python.so|{"cmdline":{"auto-complete-config":"false","backend-directory":"/opt/tritonserver/backends","min-com| |||pute-capability":"6.000000","shm-region-prefix-name":"prefix0_","default-max-batch-size":"4"}}| +-------------+-----------------------------------------------------------------+------------------------------------------------------------------------------------------------------+ I11051417.3070532561098server.cc:662] +----------------+---------+--------+ |Model|Version|Status| +----------------+---------+--------+ |ensemble|1|READY| |postprocessing|1|READY| |preprocessing|1|READY| |tensorrt_llm|1|READY| +----------------+---------+--------+ I11051417.3933182561098metrics.cc:817]CollectingmetricsforGPU0:NVIDIARTXA4000 I11051417.3935342561098metrics.cc:710]CollectingCPUmetrics I11051417.3945502561098tritonserver.cc:2458] +----------------------------------+----------------------------------------------------------------------------------------------------------------------------------------------------+ |Option|Value| +----------------------------------+----------------------------------------------------------------------------------------------------------------------------------------------------+ |server_id|triton| |server_version|2.39.0| |server_extensions|classificationsequencemodel_repositorymodel_repository(unload_dependents)schedule_policymodel_configurationsystem_shared_memorycuda_shared_| ||memorybinary_tensor_dataparametersstatisticstracelogging| |model_repository_path[0]|/work/triton_models/inflight_batcher_llm| |model_control_mode|MODE_NONE| |strict_model_config|1| |rate_limit|OFF| |pinned_memory_pool_byte_size|268435456| |cuda_memory_pool_byte_size{0}|67108864| |min_supported_compute_capability|6.0| |strict_readiness|1| |exit_timeout|30| |cache_enabled|0| +----------------------------------+----------------------------------------------------------------------------------------------------------------------------------------------------+ I11051417.4234792561098grpc_server.cc:2513]StartedGRPCInferenceServiceat0.0.0.0:8001 I11051417.4244182561098http_server.cc:4497]StartedHTTPServiceat0.0.0.0:8000 I11051417.4663782561098http_server.cc:270]StartedMetricsServiceat0.0.0.0:8002
这时也就启动了triton-inference-server,后端就是TensorRT-LLM。
可以看到LLAMA-7B-FP16精度版本,占用显存为:
+---------------------------------------------------------------------------------------+ SunNov514462023 +---------------------------------------------------------------------------------------+ |NVIDIA-SMI535.113.01DriverVersion:535.113.01CUDAVersion:12.2| |-----------------------------------------+----------------------+----------------------+ |GPUNamePersistence-M|Bus-IdDisp.A|VolatileUncorr.ECC| |FanTempPerfPwr:Usage/Cap|Memory-Usage|GPU-UtilComputeM.| |||MIGM.| |=========================================+======================+======================| |0NVIDIARTXA4000Off|0000000000.0Off|Off| |41%34CP816W/140W|15855MiB/16376MiB|0%Default| |||N/A| +-----------------------------------------+----------------------+----------------------+ +---------------------------------------------------------------------------------------+ |Processes:| |GPUGICIPIDTypeProcessnameGPUMemory| |IDIDUsage| |=======================================================================================| +---------------------------------------------------------------------------------------+
客户端
然后我们请求一下吧,先走http接口:
#执行 curl-XPOSTlocalhost:8000/v2/models/ensemble/generate-d'{"text_input":"Whatismachinelearning?","max_tokens":20,"bad_words":"","stop_words":""}' #得到返回结果 {"model_name":"ensemble","model_version":"1","sequence_end":false,"sequence_id":0,"sequence_start":false,"text_output":"⁇Whatismachinelearning?Machinelearningisasubfieldofcomputersciencethatfocusesonthedevelopmentofalgorithmsthatcanlearn"}
triton目前不支持SSE方法,想stream可以使用grpc协议,官方也提供了grpc的方法,首先安装triton客户端:
pipinstalltritonclient[all]
然后执行:
python3inflight_batcher_llm/client/inflight_batcher_llm_client.py--request-output-len200--tokenizer_dir/work/models/GPT/LLAMA/llama-7b-hf--tokenizer_typellama--streaming
请求后可以看到是一个token一个token返回的,也就是我们使用chatgpt3.5时,一个字一个字蹦的意思:
... [29953] [29941] [511] [450] [315] [4664] [457] [310] output_ids=[[0,19298,297,6641,29899,23027,3444,29892,1105,7598,16370,408,263,14547,297,3681,1434,8401,304,4517,297,29871,29896,29947,29946,29955,29889,940,3796,472,278,23933,5977,322,278,7021,16923,297,29258,265,1434,8718,670,1914,27144,297,29871,29896,29947,29945,29896,29889,940,471,263,29323,261,310,278,671,310,21837,7984,292,322,471,278,937,304,671,263,10489,380,994,29889,940,471,884,263,410,29880,928,9227,322,670,8277,5134,450,315,4664,457,310,3444,313,29896,29947,29945,29896,511,450,315,4664,457,310,12730,313,29896,29947,29945,29946,511,450,315,4664,457,310,13616,313,29896,29947,29945,29945,511,450,315,4664,457,310,9556,313,29896,29947,29945,29955,511,450,315,4664,457,310,17362,313,29896,29947,29945,29947,511,450,315,4664,457,310,12710,313,29896,29947,29945,29929,511,450,315,4664,457,310,14198,653,313,29896,29947,29953,29900,511,450,315,4664,457,310,28806,313,29896,29947,29953,29896,511,450,315,4664,457,310,27440,313,29896,29947,29953,29906,511,450,315,4664,457,310,24506,313,29896,29947,29953,29941,511,450,315,4664,457,310]] Input:Borninnorth-eastFrance,Soyertrainedasa Output:chefinParisbeforemovingtoLondonin1847.HeworkedattheReformClubandtheRoyalHotelinBrightonbeforeopeninghisownrestaurantin1851.Hewasapioneeroftheuseofsteamcookingandwasthefirsttouseagasstove.HewasalsoaprolificwriterandhisbooksincludedTheCuisineofFrance(1851),TheCuisineofItaly(1854),TheCuisineofSpain(1855),TheCuisineofGermany(1857),TheCuisineofAustria(1858),TheCuisineofRussia(1859),TheCuisineofHungary(1860),TheCuisineofSwitzerland(1861),TheCuisineofNorway(1862),TheCuisineofSweden(1863),TheCuisineof
因为开了inflight batching,其实可以同时多个请求打过来,修改request_id不要一样就可以:
#user1 python3inflight_batcher_llm/client/inflight_batcher_llm_client.py--request-output-len200--tokenizer_dir/work/models/GPT/LLAMA/llama-7b-hf--tokenizer_typellama--streaming--request_id1 #user2 python3inflight_batcher_llm/client/inflight_batcher_llm_client.py--request-output-len200--tokenizer_dir/work/models/GPT/LLAMA/llama-7b-hf--tokenizer_typellama--streaming--request_id2
至此就快速过完整个TensorRT-LLM的运行流程。
使用建议
非常建议使用docker,人生苦短。
在我们实际使用中,vllm在batch较大的场景并不慢,利用率也能打满。TensorRT-LLM和vllm的速度在某些模型上快某些模型上慢,各有优劣。
tensorrt-llm vs vllm
The most fundamental technical difference is that TensorRT-LLM relies on TensorRT ; which is a graph compiler that can produce optimised kernels for your graph. As we continue to improve TensorRT, there will be less and less needs for "manual" interventions to optimise new networks (in terms of kernels as well as taking advantage of numerical optimizations like INT4, INT8 or FP8). I hope it helps a bit.
TensorRT-LLM的特点就是借助TensorRT,TensorRT后续更新越快,支持特性越牛逼,TensorRT-LLM也就越牛逼。灵活性上,我感觉vllm和TensorRT-LLM不分上下,加上大模型的结构其实都差不多,甚至TensorRT-LLM都没有上onnx-parser,在后续更新模型上,python快速搭建模型效率也都差不了多少。
先说这么多,后续会更新些关于TensorRT-LLM和triton相关的文章。
参考
https://github.com/NVIDIA/TensorRT-LLM/issues/45
https://github.com/NVIDIA/TensorRT-LLM/tree/main
https://github.com/NVIDIA/TensorRT-LLM/issues/83
https://github.com/triton-inference-server/tensorrtllm_backend#option-2-launch-triton-server-within-the-triton-container-built-via-buildpy-script
编辑:黄飞
-
容器
+关注
关注
0文章
495浏览量
22060 -
客户端
+关注
关注
1文章
290浏览量
16679 -
python
+关注
关注
56文章
4792浏览量
84613 -
ChatGPT
+关注
关注
29文章
1558浏览量
7585
原文标题:参考
文章出处:【微信号:GiantPandaCV,微信公众号:GiantPandaCV】欢迎添加关注!文章转载请注明出处。
发布评论请先 登录
相关推荐
redhat搭建PHP运行环境LAMP的详细资料说明
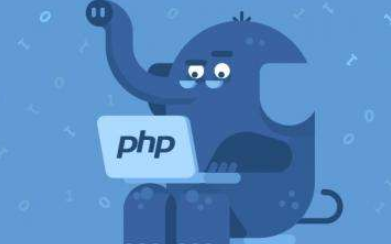
基于TensorRT完成NanoDet模型部署
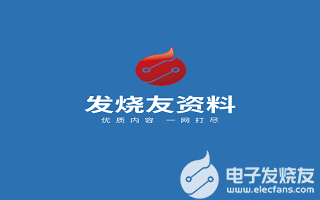
周四研讨会预告 | 注册报名 NVIDIA AI Inference Day - 大模型推理线上研讨会
现已公开发布!欢迎使用 NVIDIA TensorRT-LLM 优化大语言模型推理
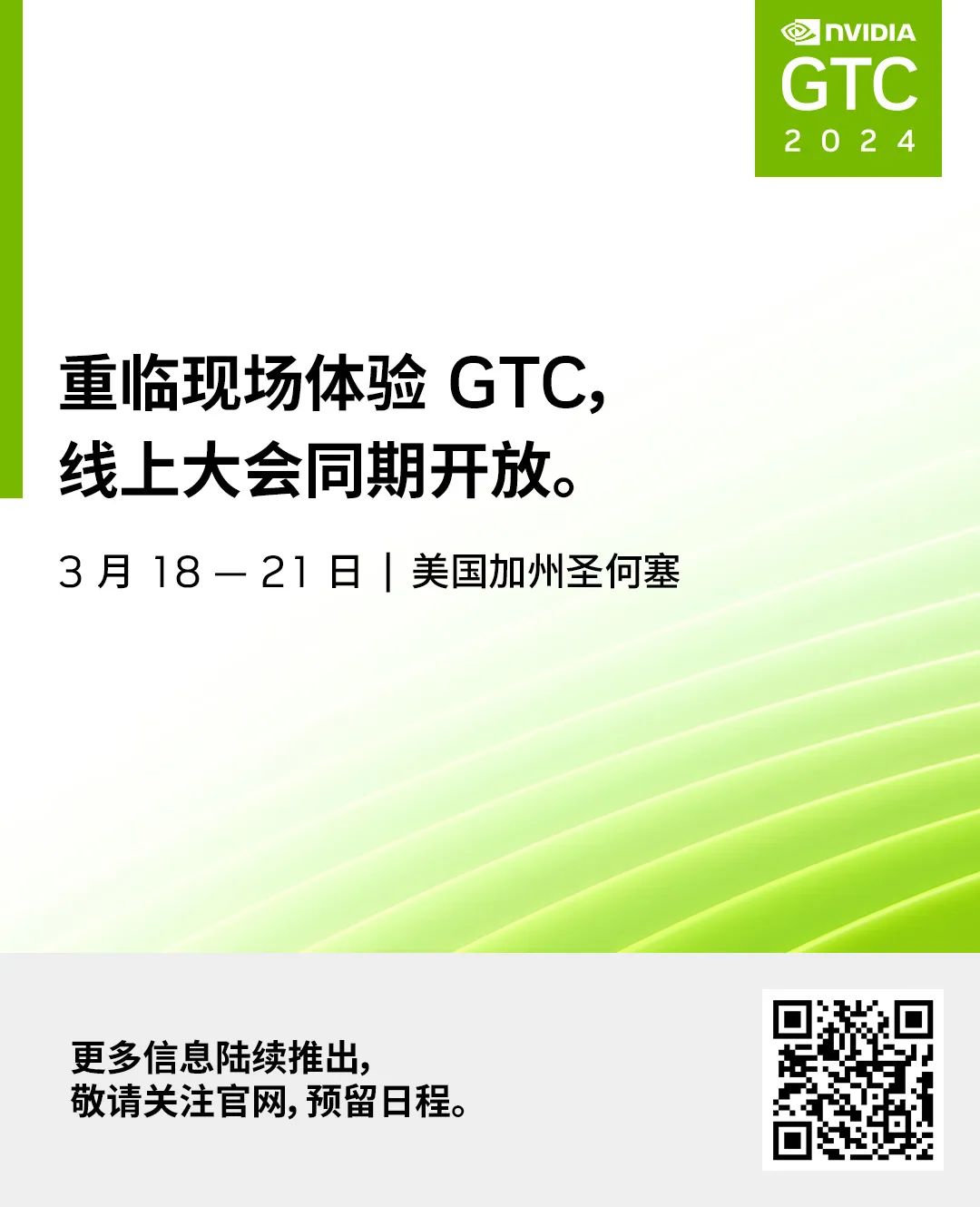
点亮未来:TensorRT-LLM 更新加速 AI 推理性能,支持在 RTX 驱动的 Windows PC 上运行新模型
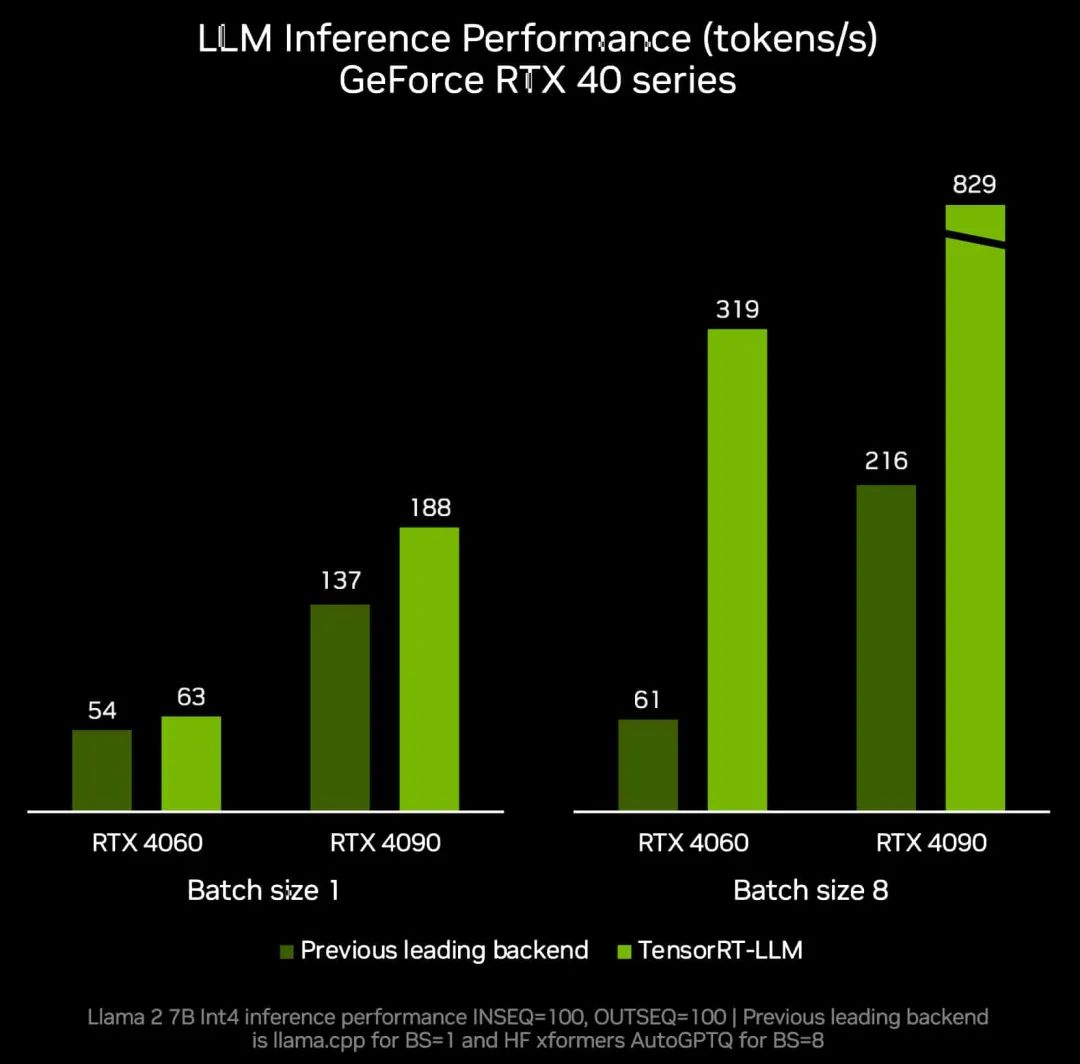
NVIDIA加速微软最新的Phi-3 Mini开源语言模型
魔搭社区借助NVIDIA TensorRT-LLM提升LLM推理效率
NVIDIA Nemotron-4 340B模型帮助开发者生成合成训练数据
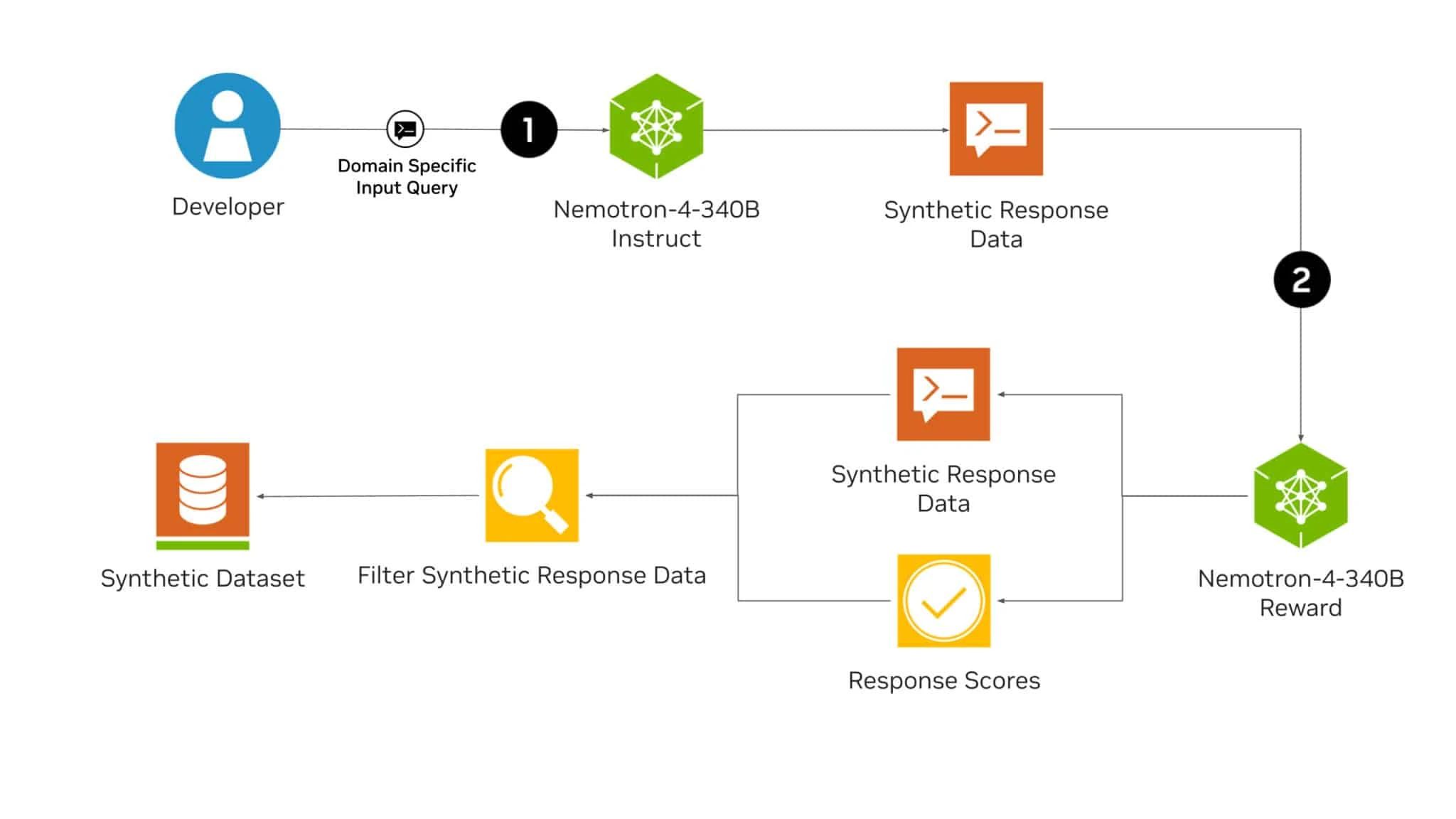
TensorRT-LLM低精度推理优化
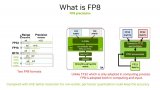
NVIDIA TensorRT-LLM Roadmap现已在GitHub上公开发布
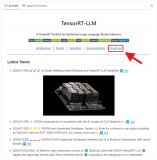
评论